Road surface crack detection method based on MobileNet-PSPNet neural network model
A neural network model and detection method technology, applied in character and pattern recognition, image analysis, image enhancement and other directions, can solve the problems of low crack detection accuracy, loss of detail information, etc., to achieve easy real-time detection, high photo quality, A wide range of effects
- Summary
- Abstract
- Description
- Claims
- Application Information
AI Technical Summary
Problems solved by technology
Method used
Image
Examples
Embodiment Construction
[0056] In order to make the purpose, technical solutions and advantages of the embodiments of the present invention clearer, the technical solutions in the embodiments of the present invention will be clearly and completely described below in conjunction with the embodiments of the present invention. Obviously, the described embodiments are part of the present invention Examples, not all examples. Based on the embodiments of the present invention, all other embodiments obtained by persons of ordinary skill in the art without making creative efforts belong to the protection scope of the present invention.
[0057] A method for detecting cracks in campus roads based on the MobileNet-PSPNet neural network model, comprising the following steps;
[0058] S1. Collect the crack image dataset, manually calibrate the label and convert it into the corresponding mask bitmap.
[0059] The crack image data set comes from the crack data set in the paper and the crack images taken manually,...
PUM
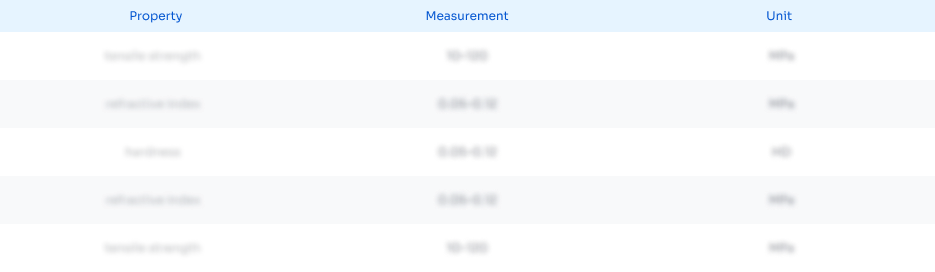
Abstract
Description
Claims
Application Information
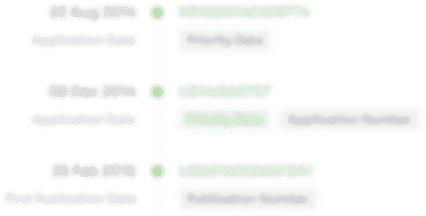
- R&D Engineer
- R&D Manager
- IP Professional
- Industry Leading Data Capabilities
- Powerful AI technology
- Patent DNA Extraction
Browse by: Latest US Patents, China's latest patents, Technical Efficacy Thesaurus, Application Domain, Technology Topic, Popular Technical Reports.
© 2024 PatSnap. All rights reserved.Legal|Privacy policy|Modern Slavery Act Transparency Statement|Sitemap|About US| Contact US: help@patsnap.com