Energy storage battery capacity prediction method, server and computer readable storage medium
A prediction method and energy storage battery technology, applied in the field of battery energy storage, can solve the problems of slow prediction speed, difficult to determine the initial parameters of the data-driven method, and inability to achieve accurate modeling, and achieve the effect of improving prediction accuracy.
- Summary
- Abstract
- Description
- Claims
- Application Information
AI Technical Summary
Problems solved by technology
Method used
Image
Examples
Embodiment 1
[0054] Please refer to Figure 1-Figure 4 A block diagram of a method for predicting the capacity of an energy storage battery provided in this application, including the following steps:
[0055] S100: Preprocessing:
[0056] Acquiring battery data, and preprocessing the battery data;
[0057] Select battery capacity degeneration feature quantity in described battery data, as the input of Elman neural network, select battery capacity as the output of Elman neural network; Described battery data is divided into training set and test set;
[0058] Specifically, the battery is an energy storage battery; the battery data is acquired by using battery charging and discharging equipment to collect data from the battery.
[0059] Specifically, performing preprocessing on the battery data includes: performing normalization and noise reduction processing on the battery data.
[0060] S200: Initialization: Initialize the cuckoo search algorithm parameters and generate N initial solutio...
Embodiment 2
[0116] Please refer to Figure 8 The functional block diagram of the server or server computer system 600 provided in this application, including a memory, a processor, and a computer program stored in the memory and operable on the processor, and the processor executes the computer program The program is to realize the steps of the energy storage battery capacity prediction method described in any one of the above.
[0117] like Figure 8 As shown, the computer system 600 includes a central processing unit (CPU) 601, which can execute according to a program stored in a read only memory (ROM) 602 or a program loaded from a storage portion into a random access memory (RAM) 603 Various appropriate actions and treatments. Various programs and data necessary for system operation are also stored in RAM 603 . The CPU 601 , ROM 602 , and RAM 603 are connected to each other through a bus 604 . An input / output (I / O) interface 605 is also connected to the bus 604 .
[0118] The fol...
Embodiment 3
[0121] The present application also provides a computer-readable storage medium, the computer-readable storage medium has a computer program, and when the computer program is executed by a processor, the steps of the energy storage battery capacity prediction method described in any one of the above are implemented.
[0122] It should be noted that the computer-readable medium shown in the present invention may be a computer-readable signal medium or a computer-readable storage medium or any combination of the above two. A computer readable storage medium may be, for example, but not limited to, an electrical, magnetic, optical, electromagnetic, infrared, or semiconductor system, apparatus, or device, or any combination thereof. More specific examples of computer-readable storage media may include, but are not limited to, electrical connections with one or more wires, portable computer diskettes, hard disks, random access memory (RAM), read-only memory (ROM), erasable Programm...
PUM
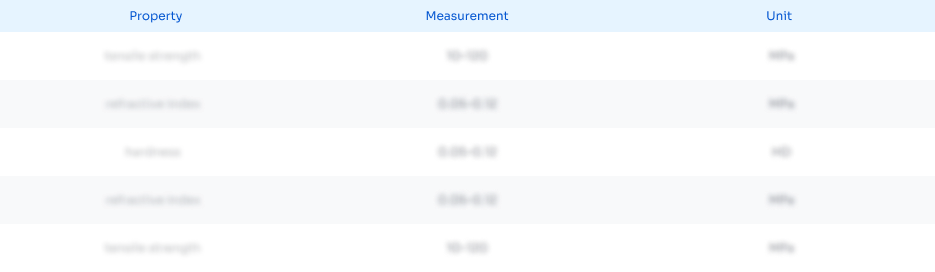
Abstract
Description
Claims
Application Information
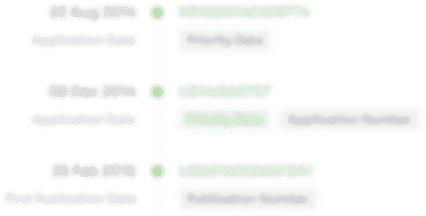
- R&D
- Intellectual Property
- Life Sciences
- Materials
- Tech Scout
- Unparalleled Data Quality
- Higher Quality Content
- 60% Fewer Hallucinations
Browse by: Latest US Patents, China's latest patents, Technical Efficacy Thesaurus, Application Domain, Technology Topic, Popular Technical Reports.
© 2025 PatSnap. All rights reserved.Legal|Privacy policy|Modern Slavery Act Transparency Statement|Sitemap|About US| Contact US: help@patsnap.com