A deep separable convolutional neural network acceleration method and accelerator
A convolutional neural network and deep convolution technology, applied in the field of depth-separable convolutional neural network acceleration methods and accelerators, to achieve efficient support, reduce access, and reduce power consumption
- Summary
- Abstract
- Description
- Claims
- Application Information
AI Technical Summary
Problems solved by technology
Method used
Image
Examples
Embodiment Construction
[0039] Specific embodiments of the present invention will be described in detail below in conjunction with the accompanying drawings. It should be understood that the specific embodiments described here are only used to illustrate and explain the present invention, and are not intended to limit the present invention.
[0040] see figure 1, which shows a depth-separable convolutional neural network acceleration method according to an embodiment of the present invention, including:
[0041] S101. Perform depth convolution on the input neurons. When performing the depth convolution calculation, the same M rows of the C input channel are independently and parallelly calculated in the three-dimensional processing unit PE array, and the same N rows of output neurons of the C channel are obtained. N
[0042] S102. Perform point convolution on the output neurons obtained by the depth convolution. When performing the point convolution calculation, each row of data of the C channel...
PUM
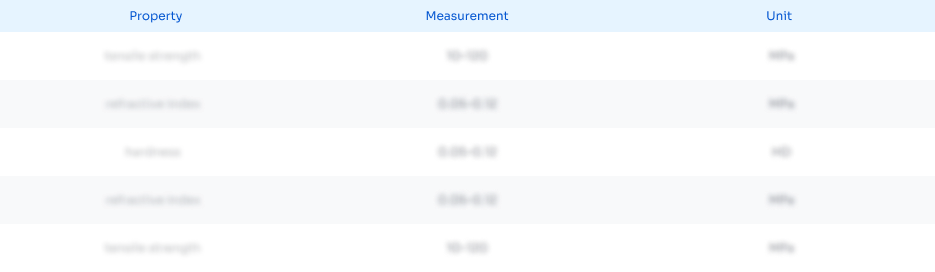
Abstract
Description
Claims
Application Information
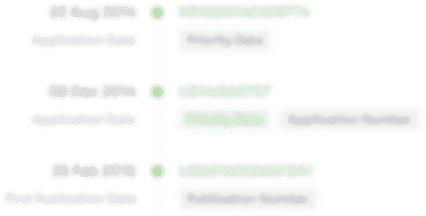
- R&D Engineer
- R&D Manager
- IP Professional
- Industry Leading Data Capabilities
- Powerful AI technology
- Patent DNA Extraction
Browse by: Latest US Patents, China's latest patents, Technical Efficacy Thesaurus, Application Domain, Technology Topic, Popular Technical Reports.
© 2024 PatSnap. All rights reserved.Legal|Privacy policy|Modern Slavery Act Transparency Statement|Sitemap|About US| Contact US: help@patsnap.com