Deep space rock image segmentation and identification method based on spiking neural network
A spiking neural network and image segmentation technology, applied in neural learning methods, biological neural network models, neural architectures, etc., to achieve the effects of strong generality and scalability, low latency, and reduced computing latency
- Summary
- Abstract
- Description
- Claims
- Application Information
AI Technical Summary
Problems solved by technology
Method used
Image
Examples
Embodiment 1
[0052] like figure 1 As shown, a rock image segmentation and recognition method based on spiking neural network, including the following steps:
[0053] S1. Preprocessing rock images in deep space environment;
[0054] S11. Using the Artificial LunarLandscape dataset image created by the Ishigami Laboratory (Space Robotics Group) of Keio University in Japan, the dataset contains 9766 synthetic images of lunar landscapes and their corresponding category segmentation (sky, ground, large-size rocks, small size rocks), while containing the bounding box for large size rocks;
[0055] S12. Preprocessing the data set. Assign the results of the model to colors to prepare for later model predictions, first use argmax to convert to category values [0,1,2,3], and then convert category values to RGB three channel values [255,0,0 ], [0,0,0], [0,0,255], [0,255,0]. The representative colors of the sky, ground, large-size rocks, and small-size rocks are red, black, blue, and green...
PUM
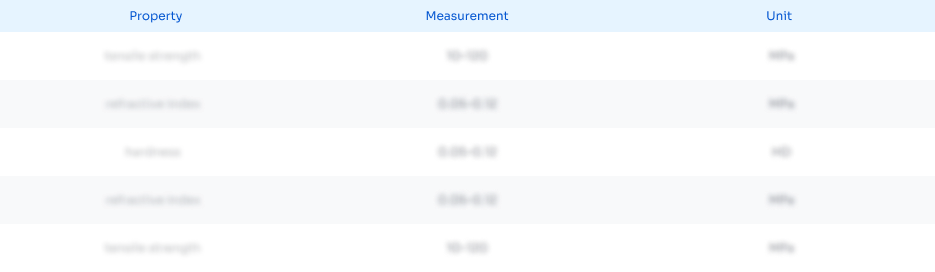
Abstract
Description
Claims
Application Information
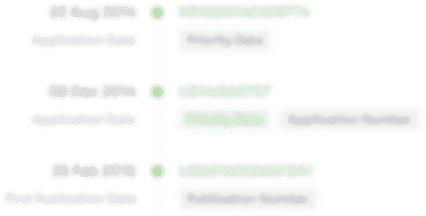
- Generate Ideas
- Intellectual Property
- Life Sciences
- Materials
- Tech Scout
- Unparalleled Data Quality
- Higher Quality Content
- 60% Fewer Hallucinations
Browse by: Latest US Patents, China's latest patents, Technical Efficacy Thesaurus, Application Domain, Technology Topic, Popular Technical Reports.
© 2025 PatSnap. All rights reserved.Legal|Privacy policy|Modern Slavery Act Transparency Statement|Sitemap|About US| Contact US: help@patsnap.com