Molecular graph generation method based on variational auto-encoder and message passing neural network
A self-encoder and neural network technology, applied in the field of molecular graph generation, can solve problems such as large chemical space and difficulty in finding target compound molecules, and achieve the effects of high unique rate, excellent efficiency and novelty rate indicators, and optimized performance advantages
- Summary
- Abstract
- Description
- Claims
- Application Information
AI Technical Summary
Problems solved by technology
Method used
Image
Examples
Embodiment 1
[0058] see Figure 1-Figure 4 , this embodiment provides a molecular graph generation method based on a variational autoencoder and a message passing neural network. In this method, this embodiment proposes a molecular generation model based on a deep generation model, which involves computer artificial intelligence and pharmaceutical molecular design The core idea is to introduce the graph neural network and property regularization into the deep generation model at the same time, which can effectively use the graph representation to capture the characteristics of the intrinsic similarity of molecules, and solve the highly complex and non-differentiable problem of molecular properties as optimization targets. .
[0059] Such as figure 1 As shown, the method includes constructing an effective drug molecule library, building a basic model for drug molecule generation, designing and implementing a multi-task reinforcement learning module, designing and implementing an anti-imita...
PUM
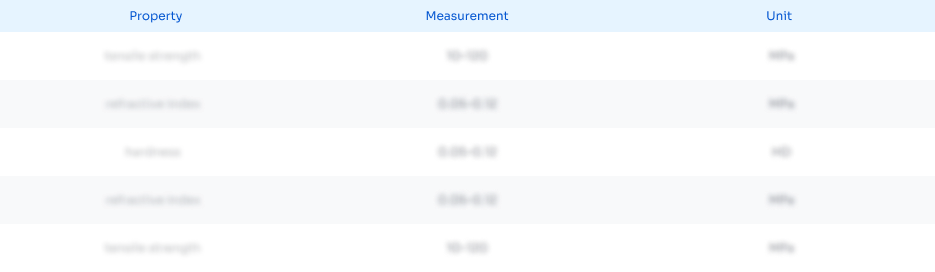
Abstract
Description
Claims
Application Information
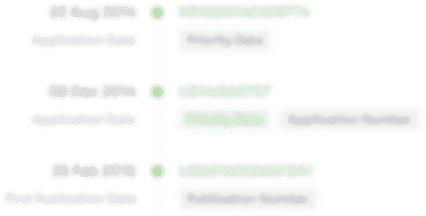
- R&D
- Intellectual Property
- Life Sciences
- Materials
- Tech Scout
- Unparalleled Data Quality
- Higher Quality Content
- 60% Fewer Hallucinations
Browse by: Latest US Patents, China's latest patents, Technical Efficacy Thesaurus, Application Domain, Technology Topic, Popular Technical Reports.
© 2025 PatSnap. All rights reserved.Legal|Privacy policy|Modern Slavery Act Transparency Statement|Sitemap|About US| Contact US: help@patsnap.com