Incremental learning method driven by task correlation
An incremental learning and correlation technology, applied in the field of image processing, can solve the problem of catastrophic forgetting of old tasks of the model
- Summary
- Abstract
- Description
- Claims
- Application Information
AI Technical Summary
Problems solved by technology
Method used
Image
Examples
Embodiment 1
[0024] Such as figure 1 As shown, the present embodiment provides a task-related incremental learning method, which includes the following steps:
[0025] image 3 Shows how a 4*4 filter in R&R-Net changes as the training progresses. Next, in a sequential fashion figure 2 Be explained:
[0026] Step 1, such as image 3 As shown in (a), before training task 1, the filter is initialized first, and all parameters in the initialized filter can be used for the training of task 1, and the labels of these parameters are marked as 1. After the initialized model learns for a certain period on the training set of task 1, it is pruned in order to cut out the redundant parameters in the model, and these redundant parameters can also be used for the learning of subsequent tasks. After pruning, it is determined which parameters in the filter are reserved for task 1 and which are available for subsequent tasks. The parameters reserved for task 1 will not be changed in the training of ...
Embodiment 2
[0036] In this embodiment, the method in the embodiment 1 is compared with the prior art and the experimental analysis is carried out.
[0037] First, we introduce the datasets used in the experiments. In total, three different datasets were used in the experiments:
[0038] (1) LIVE II: jointly established by the Department of Electrical and Computer Engineering and the Department of Psychology at the University of Texas at Austin. Contains 29 reference images and 779 distorted images, and the distorted images contain 5 types of distortion;
[0039] (2) LIVEMD: Established by the Image and Video Engineering Laboratory of the University of Texas at Austin, it contains two subsets. The first subset mixes blur distortion and JPEG distortion, the second subset mixes blur distortion and Gaussian noise, and each subset contains 225 distorted images generated from 15 original images;
[0040] (3) IVIPC_DQA: Established by the Intelligent Visual Information Processing and Communic...
PUM
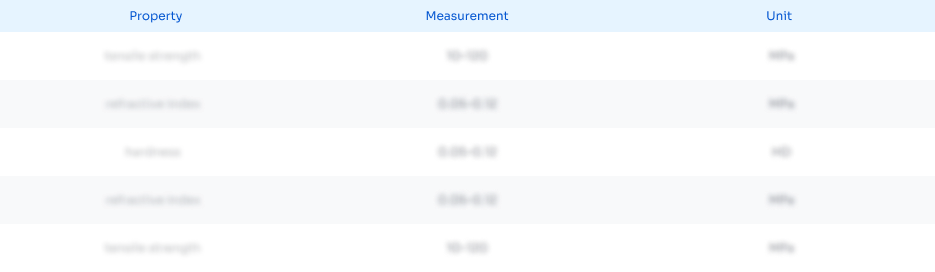
Abstract
Description
Claims
Application Information
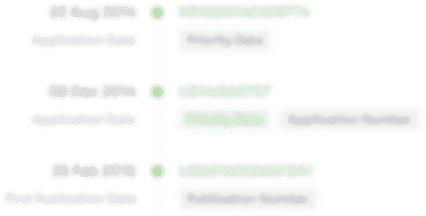
- R&D
- Intellectual Property
- Life Sciences
- Materials
- Tech Scout
- Unparalleled Data Quality
- Higher Quality Content
- 60% Fewer Hallucinations
Browse by: Latest US Patents, China's latest patents, Technical Efficacy Thesaurus, Application Domain, Technology Topic, Popular Technical Reports.
© 2025 PatSnap. All rights reserved.Legal|Privacy policy|Modern Slavery Act Transparency Statement|Sitemap|About US| Contact US: help@patsnap.com