Critical patient prognosis prediction method
A prediction method and patient technology, applied in neural learning methods, instruments, biological neural network models, etc., can solve problems such as missing data and unbalanced data, overestimation of mortality, linear relationship between predictor variables and corresponding results, etc. Accurate prognosis and the effect of reducing prediction errors
- Summary
- Abstract
- Description
- Claims
- Application Information
AI Technical Summary
Problems solved by technology
Method used
Image
Examples
Embodiment Construction
[0015] The above and other technical features and advantages of the present invention will be described in more detail below in conjunction with the accompanying drawings.
[0016] Such as figure 1 As shown, the present invention is mainly a prognostic probability calculation method based on big data of critically ill patients, which is designed to more accurately stratify the condition of critically ill patients. The method extracts and collects patient information data and laboratory test results from the hospital system, Automatically collect patient vital sign data from monitoring equipment in the intensive care unit.
[0017] Construct a prediction model based on the original big data of critically ill patients. Firstly, the characteristic factors affecting the prognosis of patients were screened out based on the training samples based on the random forest method.
[0018] Next, different statistical methods and machine learning algorithms are used to sequentially const...
PUM
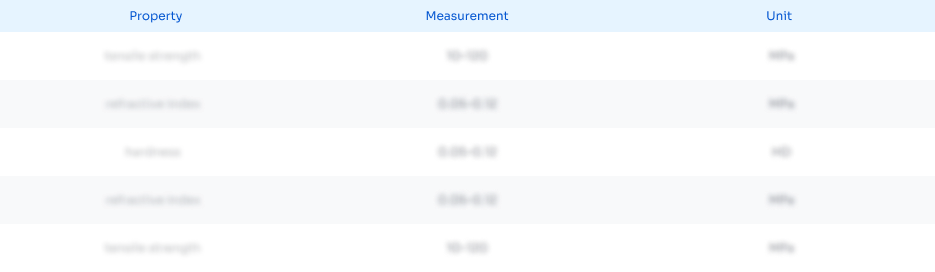
Abstract
Description
Claims
Application Information
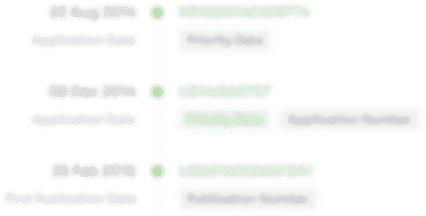
- Generate Ideas
- Intellectual Property
- Life Sciences
- Materials
- Tech Scout
- Unparalleled Data Quality
- Higher Quality Content
- 60% Fewer Hallucinations
Browse by: Latest US Patents, China's latest patents, Technical Efficacy Thesaurus, Application Domain, Technology Topic, Popular Technical Reports.
© 2025 PatSnap. All rights reserved.Legal|Privacy policy|Modern Slavery Act Transparency Statement|Sitemap|About US| Contact US: help@patsnap.com