Motor bearing fault diagnosis method based on single sample learning
A motor bearing and fault diagnosis technology, applied in the computer field, can solve the problems of the motor being impossible to run for a long time and difficult to realize, and achieve the effect of solving industrial noise, strong applicability and good characteristic information.
- Summary
- Abstract
- Description
- Claims
- Application Information
AI Technical Summary
Problems solved by technology
Method used
Image
Examples
Embodiment 1
[0043] This embodiment provides a technical solution: a single-sample learning-based motor bearing fault diagnosis method, including the following steps:
[0044] Step 1: Obtain the original one-dimensional vibration signal of the motor bearing, and study the performance of model fault diagnosis under the condition of sample scarcity and noise interference by setting different sample numbers for each type and adding different noise intensities;
[0045] Step 2: Process the motor bearing fault signal, divide it into a training set and a test set, obtain the processed samples, and select different sample sizes to train the network to judge the adaptability of the model to the sample size.
[0046]Step 3: Input the processed data set into the proposed network, and randomly select two training samples each time and send them to the Siamese network for training. Using the proposed Siamese network sub-network combined with multi-scale convolutional neural network and long short-term...
Embodiment 2
[0075] Such as Figure 1~5 As shown, this embodiment provides a technical solution: a method for diagnosing motor bearing faults based on single-sample learning, including the following steps:
[0076] Step 1. Obtain the original one-dimensional vibration signal of the motor bearing, and study the performance of model fault diagnosis under the condition of sample scarcity and noise interference by setting different sample numbers for each type and adding different noise intensities;
[0077] Step 2: Process the motor bearing fault signal, divide it into a training set and a test set, obtain the processed samples, and select different numbers of training samples for training the model.
[0078] Step 3: Input the processed dataset into the Siamese network, which contains two sub-networks with weight sharing between the two sub-networks. Two training samples are randomly selected each time and sent to the Siamese network for training. The proposed sub-network structure is used ...
PUM
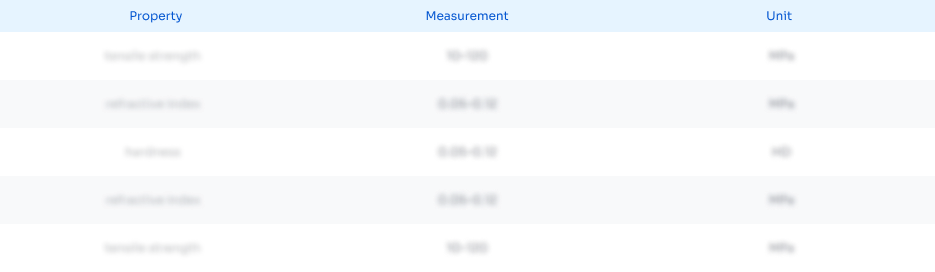
Abstract
Description
Claims
Application Information
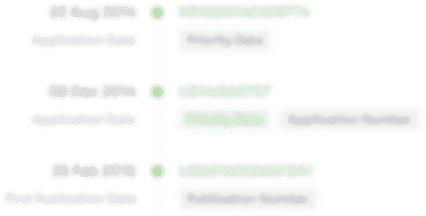
- R&D
- Intellectual Property
- Life Sciences
- Materials
- Tech Scout
- Unparalleled Data Quality
- Higher Quality Content
- 60% Fewer Hallucinations
Browse by: Latest US Patents, China's latest patents, Technical Efficacy Thesaurus, Application Domain, Technology Topic, Popular Technical Reports.
© 2025 PatSnap. All rights reserved.Legal|Privacy policy|Modern Slavery Act Transparency Statement|Sitemap|About US| Contact US: help@patsnap.com