Anomaly detection algorithm configuration method and device, electronic equipment and storage medium
A technology of abnormal detection and configuration method, applied in the field of automobile production lines, can solve the problems of multiple manpower and material resources, consumption, high cost of automobile production lines, etc., and achieve the effect of reducing manpower and material resources and reducing costs.
- Summary
- Abstract
- Description
- Claims
- Application Information
AI Technical Summary
Problems solved by technology
Method used
Image
Examples
Embodiment 1
[0130] figure 1 It is a flowchart of a method 100 for configuring an anomaly detection algorithm provided in Embodiment 1 of the present application, as shown in figure 1 As shown, the configuration method 100 of the anomaly detection algorithm includes the following steps:
[0131] 101. Obtain the operating data of the device.
[0132] The automobile production line includes multiple devices that require predictive maintenance. For each device that requires predictive maintenance, a corresponding anomaly detection algorithm is configured, but the parameters of the anomaly detection algorithm may not be suitable for the actual operating conditions of the equipment. This requires tuning the parameters of the anomaly detection algorithm. Equipment in an automotive production line that requires predictive maintenance can be a single component, such as a bearing, or an assembly of multiple components, such as a high-speed lift.
[0133] For the equipment that needs predictive m...
Embodiment 2
[0144] On the basis of the configuration method of the abnormality detection algorithm provided in Embodiment 1, if it is determined that the abnormality detection frequency of the equipment detected by the abnormality detection algorithm is stable, an alarm message can be sent to the user after the abnormality detection algorithm detects that the equipment is abnormal, and the The user further confirms whether the device is indeed abnormal, and then adjusts the parameters of the abnormality detection algorithm according to the user's confirmation result, thereby improving the accuracy of the abnormality detection algorithm for detecting device abnormalities.
[0145] figure 2 It is a schematic diagram of a parameter adjustment method 200 of an abnormality detection algorithm provided in Embodiment 2 of the present application, as shown in figure 2 As shown, after the data processing program 202 obtains the operating data of the equipment according to the configuration infor...
Embodiment 3
[0155] exist figure 1 On the basis of the configuration method 100 of the abnormality detection algorithm shown, step 104 judges whether the abnormality frequency of the equipment detected by the abnormality detection algorithm is stable, and can detect the difference in the abnormality frequency of the equipment according to the abnormality detection algorithm in different detection periods, To determine whether the abnormal frequency detected by the abnormality detection algorithm is stable. image 3 It is a flow chart of a method 300 for judging the stability of abnormal occurrence frequency provided by Embodiment 3 of the present application, as shown in image 3 As shown, the abnormal occurrence frequency stability judgment method 300 includes the following steps:
[0156] 301. Calculate a first abnormality frequency according to an abnormality detection result, where the first abnormality frequency is used to represent a frequency at which an abnormality detection algor...
PUM
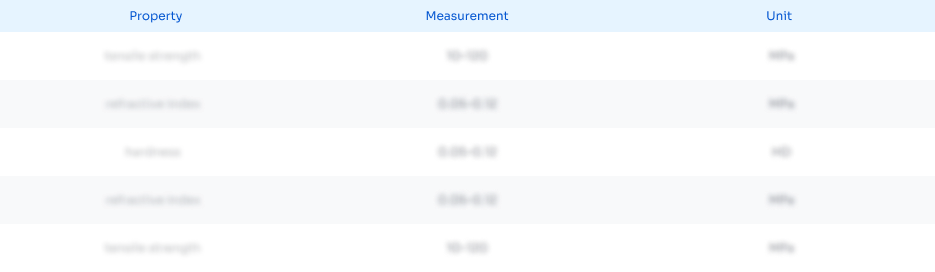
Abstract
Description
Claims
Application Information
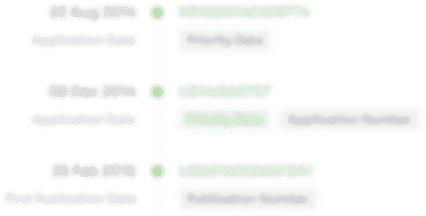
- Generate Ideas
- Intellectual Property
- Life Sciences
- Materials
- Tech Scout
- Unparalleled Data Quality
- Higher Quality Content
- 60% Fewer Hallucinations
Browse by: Latest US Patents, China's latest patents, Technical Efficacy Thesaurus, Application Domain, Technology Topic, Popular Technical Reports.
© 2025 PatSnap. All rights reserved.Legal|Privacy policy|Modern Slavery Act Transparency Statement|Sitemap|About US| Contact US: help@patsnap.com