Information physical system safety control method based on deep reinforcement learning
A cyber-physical system and reinforcement learning technology, which is applied in the field of security control of cyber-physical systems based on deep reinforcement learning, can solve problems such as poor control performance of security control strategies, and achieve improved control performance, strong robustness, and guaranteed stability Effect
- Summary
- Abstract
- Description
- Claims
- Application Information
AI Technical Summary
Problems solved by technology
Method used
Image
Examples
specific Embodiment approach 1
[0071] Specific implementation mode 1. Combination figure 1 This embodiment will be described. A cyber-physical system security control method based on deep reinforcement learning described in this embodiment, the method specifically includes the following steps:
[0072] Step 1. Establish a cyber-physical system model under the false data injection attack of the actuator;
[0073] Step 2. Describe the cyber-physical system model under the false data injection attack of the actuator established in step 1 as a Markov decision process;
[0074] Step 3: Build a deep neural network, and output a decision strategy for the Markov decision process based on the built deep neural network.
specific Embodiment approach 2
[0075] Specific implementation mode two: the difference between this implementation mode and specific implementation mode one is that the specific process of the step one is:
[0076] Step 11. Ideally, the dynamic equation of the cyber-physical system model is:
[0077]
[0078] in, represents the state vector of the cyber-physical system, represents the field of real numbers, n x Indicates the dimension of the state vector x, Indicates the control signal to be designed, n u Indicates the dimension of the control signal u, is the first-order derivative of x, and f(·) represents the generalized function mapping;
[0079] Step 12. Discretize the cyber-physical system model in step 11 based on the Euler method to obtain a discretized cyber-physical system model:
[0080] x(k+1)=(f(x(k),u(k)))Δt+x(k)
[0081] Among them, x(k) represents the state vector of the discretized cyber-physical system at time k, u(k) represents the control signal at time k, Δt represents the...
specific Embodiment approach 3
[0092] Embodiment 3: This embodiment is different from Embodiment 1 or 2 in that the attack distribution matrix Γ is a diagonal matrix, and the values of the diagonal elements are all 0 or 1. If the i-th actuator is attacked , then the i-th diagonal element of the attack distribution matrix Γ (that is, the element in the i-th row and i-column of the diagonal matrix, corresponding to the i-th actuator) takes the value 1, otherwise, the i-th diagonal element takes the value 0 .
[0093] Other steps and parameters are the same as those in Embodiment 1 or Embodiment 2.
PUM
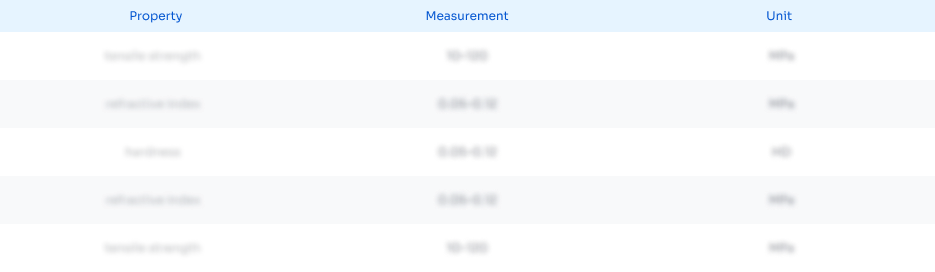
Abstract
Description
Claims
Application Information
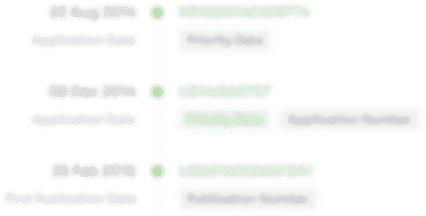
- R&D Engineer
- R&D Manager
- IP Professional
- Industry Leading Data Capabilities
- Powerful AI technology
- Patent DNA Extraction
Browse by: Latest US Patents, China's latest patents, Technical Efficacy Thesaurus, Application Domain, Technology Topic, Popular Technical Reports.
© 2024 PatSnap. All rights reserved.Legal|Privacy policy|Modern Slavery Act Transparency Statement|Sitemap|About US| Contact US: help@patsnap.com