STO-BTCN thermal error prediction model modeling method and transfer learning method thereof
A predictive model and modeling method technology, applied in neural learning methods, biological neural network models, design optimization/simulation, etc., can solve problems such as difficult to improve prediction accuracy, improvement is not always effective, etc., to achieve excellent timing The effect of relying on capture ability, easy training, and simple recursive structure
- Summary
- Abstract
- Description
- Claims
- Application Information
AI Technical Summary
Problems solved by technology
Method used
Image
Examples
Embodiment Construction
[0073] The present invention will be further described below in conjunction with the accompanying drawings and specific embodiments, so that those skilled in the art can better understand the present invention and implement it, but the examples given are not intended to limit the present invention.
[0074] Such as figure 1 As shown, the flow chart of the embodiment of the STO-BTCN thermal error prediction model modeling method of the present invention. The STO-BTCN thermal error prediction model modeling method of the present embodiment comprises the following steps:
[0075] 1) Initialize the parameters of the tern optimization algorithm (STO), randomly generate the initial position of the tern; judge whether the initial position of the tern exceeds the preset range, if so, change the initial position of the tern to the boundary; if not, then Keep the initial position of the tern unchanged. Inspired by the foraging behavior of terns in nature, the STO optimization algorith...
PUM
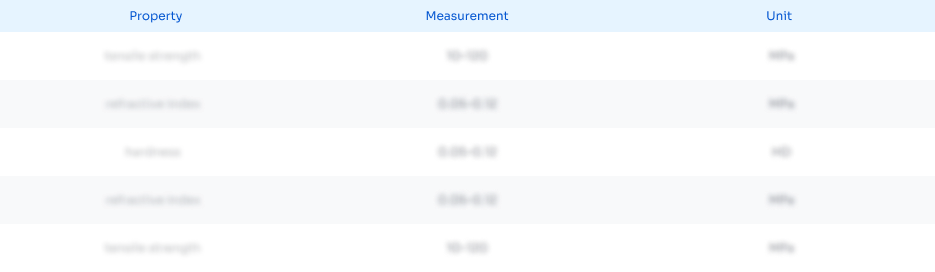
Abstract
Description
Claims
Application Information
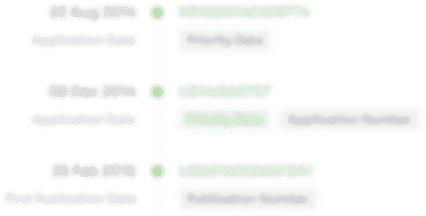
- R&D Engineer
- R&D Manager
- IP Professional
- Industry Leading Data Capabilities
- Powerful AI technology
- Patent DNA Extraction
Browse by: Latest US Patents, China's latest patents, Technical Efficacy Thesaurus, Application Domain, Technology Topic, Popular Technical Reports.
© 2024 PatSnap. All rights reserved.Legal|Privacy policy|Modern Slavery Act Transparency Statement|Sitemap|About US| Contact US: help@patsnap.com