Power system robust state estimation method and system based on deep learning
A state estimation and power system technology, applied in computing, electrical digital data processing, design optimization/simulation, etc., can solve problems such as poor timeliness and low prediction accuracy, and achieve the effects of reducing influence, improving filtering effect, and improving accuracy
- Summary
- Abstract
- Description
- Claims
- Application Information
AI Technical Summary
Problems solved by technology
Method used
Image
Examples
Embodiment 1
[0039] Such as figure 1 and figure 2 As shown, Embodiment 1 of the present invention provides a robust state estimation method for power systems based on deep learning, including the following steps:
[0040] Step S1: Historical data training, learning input historical data based on ATT-CNN-LSTM and KRRSE models, establishing and saving prediction and filtering models.
[0041] Step S2: Determine the abnormal and missing data. For the measurement data of node n, the SVM classification is used to judge whether there is missing or abnormal data in the data set, including:
[0042] Step S201: When the SVM determines that the data is normal, import the data into the KRRSE filter model saved in step S1, process the data and export the state estimation result;
[0043] Step S202: When the SVM determines that the data is missing or abnormal, import the data into the ATT-CNN-LSTM model pre-built in step S1 for fast prediction, and output the estimation result.
[0044] Specificall...
Embodiment 2
[0151] Embodiment 2 of the present invention provides a robust state estimation system for power systems based on deep learning, including:
[0152] The data acquisition module is configured to: acquire the operating parameter data of the power system;
[0153] The data judging module is configured to: judge the abnormality and / or absence of the acquired operation parameter data;
[0154] The first estimation module is configured to: obtain a robust state estimation result according to the obtained operating parameter data and the kernel ridge regression model when there is an abnormality and / or absence;
[0155] The second estimation module is configured to: when there is an abnormality and / or loss, the convolutional neural network of the attention mechanism is used to perform weight screening on the operating parameter data, and a robust state estimation result is obtained according to the long-short-term memory neural network.
[0156] The working method of the system is t...
Embodiment 3
[0158] Embodiment 3 of the present invention provides a computer-readable storage medium, on which a program is stored. When the program is executed by a processor, the method for robust state estimation of a power system based on deep learning as described in Embodiment 1 of the present invention is implemented. A step of.
PUM
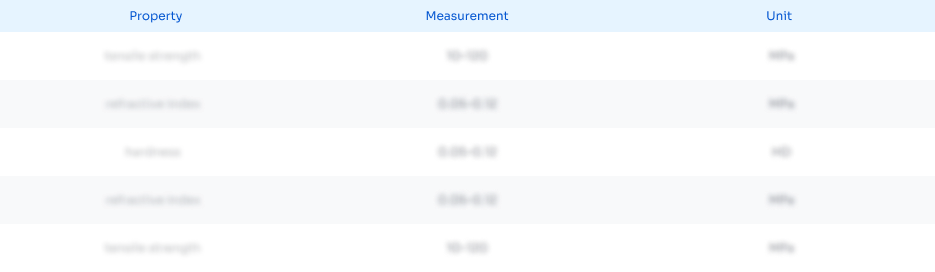
Abstract
Description
Claims
Application Information
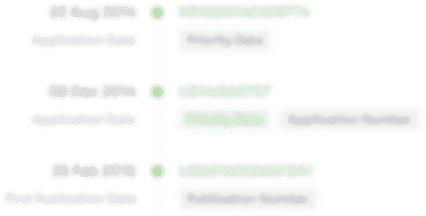
- R&D
- Intellectual Property
- Life Sciences
- Materials
- Tech Scout
- Unparalleled Data Quality
- Higher Quality Content
- 60% Fewer Hallucinations
Browse by: Latest US Patents, China's latest patents, Technical Efficacy Thesaurus, Application Domain, Technology Topic, Popular Technical Reports.
© 2025 PatSnap. All rights reserved.Legal|Privacy policy|Modern Slavery Act Transparency Statement|Sitemap|About US| Contact US: help@patsnap.com