Adaptive multi-channel graph convolutional network of joint graph contrast learning
A convolutional network and multi-channel technology, applied in the field of graph-based convolutional network and graph comparison learning, can solve problems such as easy loss of information, and achieve the effect of improving classification performance
- Summary
- Abstract
- Description
- Claims
- Application Information
AI Technical Summary
Problems solved by technology
Method used
Image
Examples
Embodiment Construction
[0024] Below in conjunction with accompanying drawing, the present invention will be further described.
[0025] The overall frame diagram of the present invention is as figure 1 shown. figure 2 Shown is a flowchart of the method implementation. image 3 Shown is the process of constructing functional brain network and structural connection brain network map in each window. Figure 4 It uses the attention mechanism to adaptively fuse the features extracted by multi-channel GCN. Figure 5 A comparative strategy for optimizing features between different modalities is demonstrated.
[0026] The following is based on figure 2 The illustrated implementation framework illustrates the specific implementation process of an adaptive multi-channel graph convolutional network method for joint graph comparison learning provided above in the present invention, and is not limited to multi-modal brain network learning in the actual application process.
[0027] First, we use overlappi...
PUM
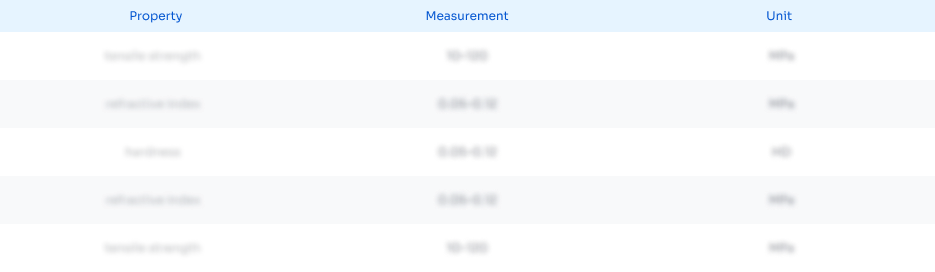
Abstract
Description
Claims
Application Information
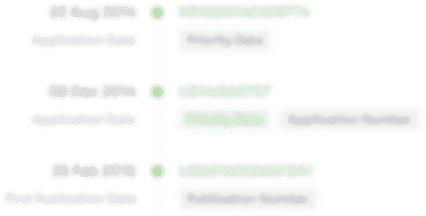
- R&D Engineer
- R&D Manager
- IP Professional
- Industry Leading Data Capabilities
- Powerful AI technology
- Patent DNA Extraction
Browse by: Latest US Patents, China's latest patents, Technical Efficacy Thesaurus, Application Domain, Technology Topic, Popular Technical Reports.
© 2024 PatSnap. All rights reserved.Legal|Privacy policy|Modern Slavery Act Transparency Statement|Sitemap|About US| Contact US: help@patsnap.com