Multi-mode two-stage unsupervised video anomaly detection method
An anomaly detection and unsupervised technology, applied in neural learning methods, biological neural network models, image analysis, etc., can solve the problems of high missed detection rate and insufficient robustness, achieve enhanced accuracy, reduce missed detection probability, Enhanced Robustness Effects
- Summary
- Abstract
- Description
- Claims
- Application Information
AI Technical Summary
Problems solved by technology
Method used
Image
Examples
Embodiment Construction
[0039] Embodiments of the present invention are described below through specific examples, and those skilled in the art can easily understand other advantages and effects of the present invention from the content disclosed in this specification. The present invention can also be implemented or applied through other different specific implementation modes, and various modifications or changes can be made to the details in this specification based on different viewpoints and applications without departing from the spirit of the present invention. It should be noted that, in the case of no conflict, the following embodiments and features in the embodiments can be combined with each other.
[0040] Such as figure 1 As shown, the embodiment of the present invention includes a multimodal two-stage unsupervised abnormal video detection method. In the first stage, a multi-scale memory-enhanced autoencoder network is designed, and the network is used to learn the image features and op...
PUM
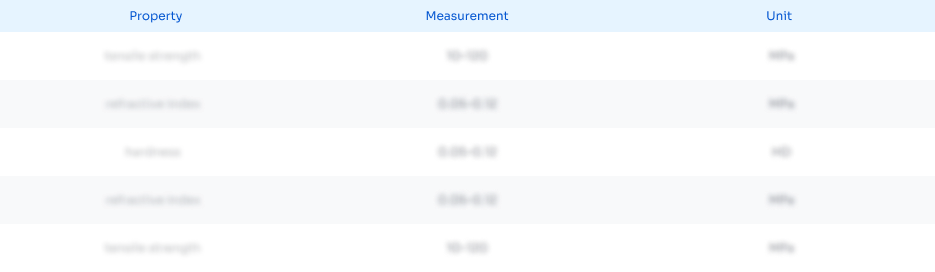
Abstract
Description
Claims
Application Information
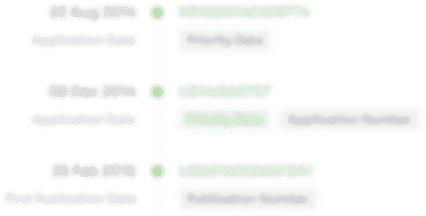
- R&D Engineer
- R&D Manager
- IP Professional
- Industry Leading Data Capabilities
- Powerful AI technology
- Patent DNA Extraction
Browse by: Latest US Patents, China's latest patents, Technical Efficacy Thesaurus, Application Domain, Technology Topic, Popular Technical Reports.
© 2024 PatSnap. All rights reserved.Legal|Privacy policy|Modern Slavery Act Transparency Statement|Sitemap|About US| Contact US: help@patsnap.com