Compressor blade crack fault detection method
A technology for compressor blades and fault detection, which is applied in neural learning methods, measuring devices, computer parts, etc., can solve problems such as affecting the accuracy of fault detection, prone to errors, and time-consuming, so as to achieve high-accuracy detection, improve Accuracy, the effect of reducing the number of
- Summary
- Abstract
- Description
- Claims
- Application Information
AI Technical Summary
Problems solved by technology
Method used
Image
Examples
Embodiment Construction
[0041] The following description is merely exemplary in nature and is not intended to limit the disclosure, application, or uses. It should be understood that throughout the drawings, corresponding reference numerals indicate like or corresponding parts and features.
[0042] like figure 1 As shown, the compressor blade crack fault detection method according to the embodiment of the present invention includes the following steps:
[0043] Step 1: Collect two-channel acoustic emission signals at the compressor air outlet, and divide them into two-channel training samples and two-channel test samples;
[0044] Step 2: perform feature extraction on the two-channel training samples, including acoustic emission features, time-domain features, frequency-domain features, and spectral centroid energy migration features;
[0045] Step 3: Select features using a hybrid feature selection method to establish an optimal feature subset;
[0046] Step 4: Extract the features of the two-ch...
PUM
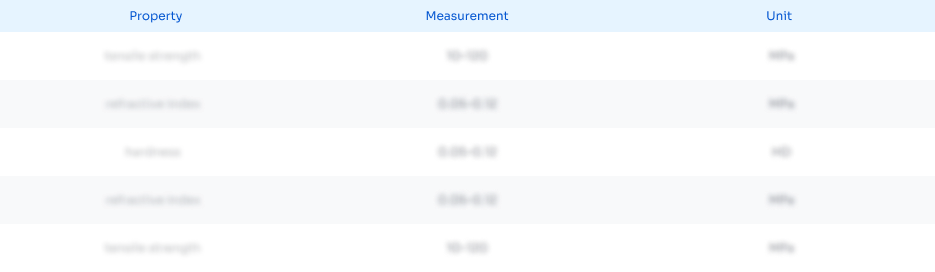
Abstract
Description
Claims
Application Information
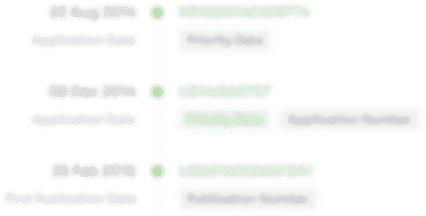
- R&D Engineer
- R&D Manager
- IP Professional
- Industry Leading Data Capabilities
- Powerful AI technology
- Patent DNA Extraction
Browse by: Latest US Patents, China's latest patents, Technical Efficacy Thesaurus, Application Domain, Technology Topic, Popular Technical Reports.
© 2024 PatSnap. All rights reserved.Legal|Privacy policy|Modern Slavery Act Transparency Statement|Sitemap|About US| Contact US: help@patsnap.com