Workshop scheduling method, device and system based on deep reinforcement learning
A technology of reinforcement learning and workshop scheduling, applied in control/regulation systems, manufacturing computing systems, general control systems, etc., can solve the problems of low efficiency and poor compatibility of dynamic online scheduling methods, and achieve high decision-making efficiency, strong compatibility, The effect of great application prospects
- Summary
- Abstract
- Description
- Claims
- Application Information
AI Technical Summary
Problems solved by technology
Method used
Image
Examples
Embodiment Construction
[0045] In order to make the objectives, technical solutions and advantages of the present invention clearer, the present invention will be further described in detail below with reference to the accompanying drawings and embodiments. It should be understood that the specific embodiments described herein are only used to explain the present invention, but not to limit the present invention. In addition, the technical features involved in the various embodiments of the present invention described below can be combined with each other as long as they do not conflict with each other.
[0046] Deep Reinforcement Learning (DRL) adds a neural network to approximate the value function based on reinforcement learning, so that reinforcement learning can solve large-scale and continuous state space problems. DRL is a method that does not require the establishment of mathematical models to solve problems only by interacting with the environment. DRL can be regarded as an agent, and the p...
PUM
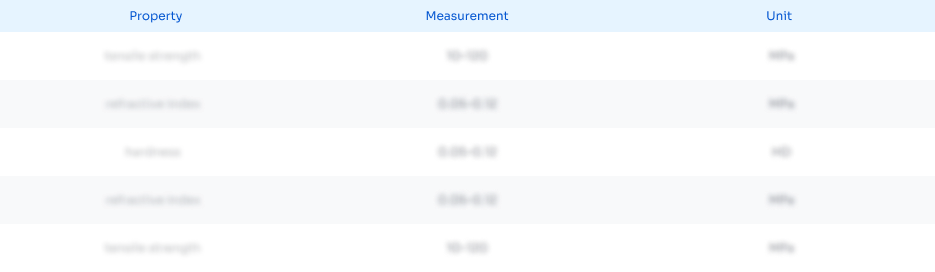
Abstract
Description
Claims
Application Information
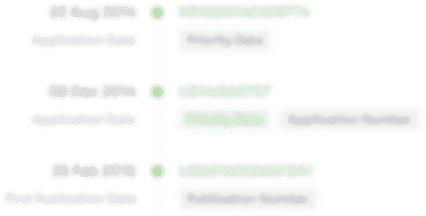
- R&D Engineer
- R&D Manager
- IP Professional
- Industry Leading Data Capabilities
- Powerful AI technology
- Patent DNA Extraction
Browse by: Latest US Patents, China's latest patents, Technical Efficacy Thesaurus, Application Domain, Technology Topic, Popular Technical Reports.
© 2024 PatSnap. All rights reserved.Legal|Privacy policy|Modern Slavery Act Transparency Statement|Sitemap|About US| Contact US: help@patsnap.com