Gradient-based adversarial sample generation method and system
An adversarial sample and gradient technology, applied in the fields of computer vision and deep learning, can solve the problems of poor transferability and high noise visibility, and achieve the effect of improving the attack success rate, reducing the noise size, and reducing loss.
- Summary
- Abstract
- Description
- Claims
- Application Information
AI Technical Summary
Problems solved by technology
Method used
Image
Examples
Embodiment 1
[0063] This embodiment discloses a gradient-based adversarial sample generation method, which is suitable for both white-box attacks and black-box attacks. Among them, the white-box attack refers to all the information of the neural network model to be attacked, such as structure, parameters, training process and training data, when the attack is known. Black-box attack means that only limited information of the neural network model to be attacked, such as confidence and label, is known during the attack.
[0064] like figure 1 As shown, the gradient-based adversarial sample generation method includes the following steps:
[0065] S1. Obtain the original image sample and the neural network model to be attacked.
[0066] Specifically, the original image sample dataset used in this experiment is the ImageNet-compatible dataset, which is the official dataset of the confrontation competition held at the 2017 Conference on Advances in Neural Information Processing Systems, includ...
Embodiment 2
[0093] This embodiment discloses a gradient-based adversarial sample generation system, such as Image 6 As shown, it includes the following functional modules:
[0094] The acquisition module is used to acquire the original image samples and the neural network model to be attacked. The image format of the original image sample is .png or .jpg format, and the format of the neural network model file is .ckpt format.
[0095]The loss calculation module inputs the original image sample into the neural network model, and obtains the loss information of the original image sample according to the cross-entropy loss function. The input of the loss calculation module is the original image sample obtained by the obtaining module and the neural network model to be attacked, and the output is loss information. Among them, the cross entropy loss function in tensorflow is tf.nn.softmax_cross_entropy_with_logits().
[0096] Specifically, the loss calculation module includes a normal loss...
PUM
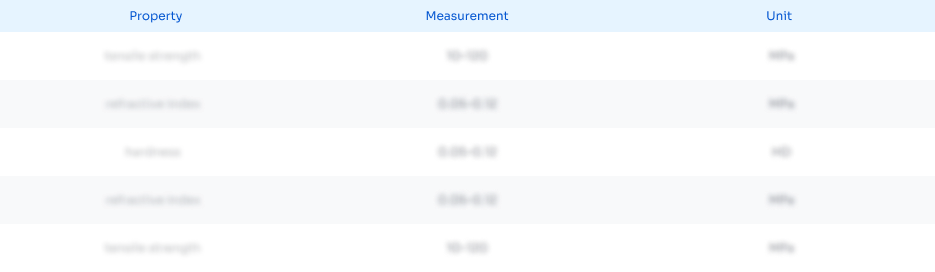
Abstract
Description
Claims
Application Information
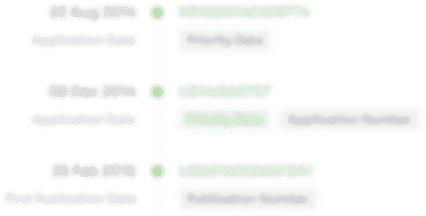
- R&D Engineer
- R&D Manager
- IP Professional
- Industry Leading Data Capabilities
- Powerful AI technology
- Patent DNA Extraction
Browse by: Latest US Patents, China's latest patents, Technical Efficacy Thesaurus, Application Domain, Technology Topic, Popular Technical Reports.
© 2024 PatSnap. All rights reserved.Legal|Privacy policy|Modern Slavery Act Transparency Statement|Sitemap|About US| Contact US: help@patsnap.com