Bearing fault detection and classification integrated method based on representation learning
A fault detection and fault classification technology, applied in the interdisciplinary field, can solve the problems of difficult bearing fault samples and label limitations, and achieve the effect of improving the reconstruction effect and the accuracy rate.
- Summary
- Abstract
- Description
- Claims
- Application Information
AI Technical Summary
Problems solved by technology
Method used
Image
Examples
specific Embodiment approach 1
[0038] Embodiment 1: The specific process of an integrated method for bearing fault detection and classification based on representation learning in this embodiment is as follows:
[0039] In the present invention, the neural network is used to represent the learning method;
[0040] Step 1. Extract the vibration data of the bearing:
[0041] Through the sensor equipment on the bearing housing, such as wireless sensors, etc., the vibration data of the bearing during the working process is collected as the input of the integrated method of bearing fault detection and classification based on representation learning;
[0042] The vibration data includes health data and fault data (10% of the fault data is used for offline training);
[0043] Step 2. Feature extraction:
[0044] In order to better reflect the healthy state of the bearing during the working process, it is considered to extract the characteristics of the vibration data of the bearing during the working process fro...
specific Embodiment approach 2
[0059] Embodiment 2: The difference between this embodiment and Embodiment 1 is that in the feature extraction in step 2: in order to better reflect the health status of the bearing during the working process, it is considered to extract the bearing from the perspectives of the time domain and the frequency domain. The characteristics of the vibration data during the working process, thus serving as the input of the neural network;
[0060] The characteristics of the vibration data include the characteristics of the health data and the characteristics of the fault data;
[0061] The specific process is:
[0062] In order to better reflect the healthy state of the bearing during the working process, the present invention considers extracting the characteristics of the vibration data generated by the bearing during the working phase from the time domain, frequency domain and these two perspectives, and thus serves as the input of the neural network. As a commonly used signal fe...
specific Embodiment approach 3
[0084] Embodiment 3: The difference between this embodiment and Embodiment 1 or 2 is that a fault detection neural network is built in step 3: the fault detection neural network includes an encoder, a noise introduction, a self-attention mechanism layer, and a decoder;
[0085] In order to reduce the influence of noise signal on sample reconstruction, and assign different weights to different bottleneck layer neurons, so as to effectively realize fault detection, the present invention proposes a self-attention mechanism based on bottleneck layer neurons to correct and denoise Neural network of Modified denoising auto-encoder with Self-attention mechanism for bottleneck layer, MDAE-SAMB. The MDAE-SAMB network is trained by using only the features obtained by feature extraction from the vibration data of the bearing in a healthy state. The MDAE-SAMB structure is mainly composed of four parts: encoder, noise introduction, self-attention mechanism layer and decoder.
[0086] The ...
PUM
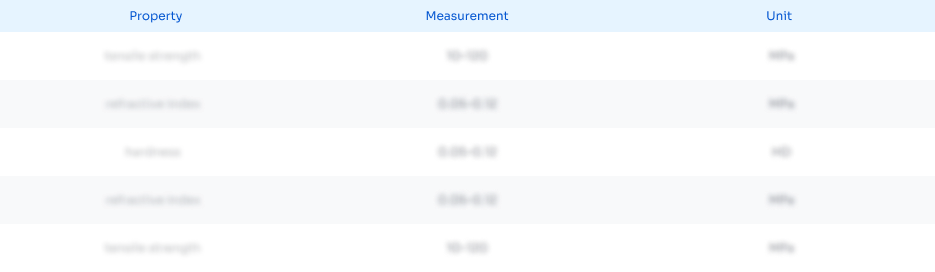
Abstract
Description
Claims
Application Information
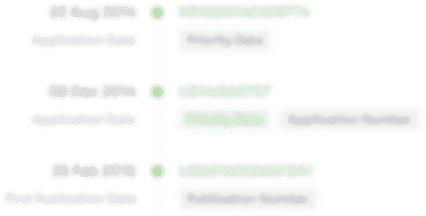
- R&D
- Intellectual Property
- Life Sciences
- Materials
- Tech Scout
- Unparalleled Data Quality
- Higher Quality Content
- 60% Fewer Hallucinations
Browse by: Latest US Patents, China's latest patents, Technical Efficacy Thesaurus, Application Domain, Technology Topic, Popular Technical Reports.
© 2025 PatSnap. All rights reserved.Legal|Privacy policy|Modern Slavery Act Transparency Statement|Sitemap|About US| Contact US: help@patsnap.com