Non-uniform noise removal method based on stepped multi-scale convolutional neural network
A convolutional neural network, non-uniform noise technology, applied in strip, plane non-uniform noise image denoising, point field, can solve problems such as reducing the amount of calculation, reducing the generalization ability of the model, and overfitting the model, Achieve the effect of improving robustness and accuracy, preventing deep network degradation, and optimizing learning ability
- Summary
- Abstract
- Description
- Claims
- Application Information
AI Technical Summary
Problems solved by technology
Method used
Image
Examples
Embodiment Construction
[0030] In order to more clearly understand the above objects, features and advantages of the present invention, the present invention will be further described below with reference to the accompanying drawings and embodiments. Numerous specific details are set forth in the following description to facilitate a full understanding of the present invention, however, the present invention may also be practiced in other ways than those described herein, and therefore, the present invention is not limited to the specific embodiments disclosed below.
[0031] The invention proposes a non-uniform noise removal method based on a stepped multi-scale convolutional neural network, realizes the noise recognition at the pixel level of the image according to the full convolutional neural network (FCN), abandons the pooling and deconvolution layers, and uses the mean square Error (MSE) is the objective function, and through adaptive gradient optimization, the end-to-end mapping of noisy images...
PUM
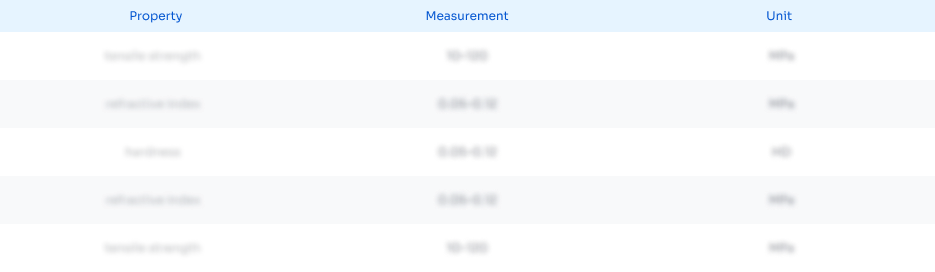
Abstract
Description
Claims
Application Information
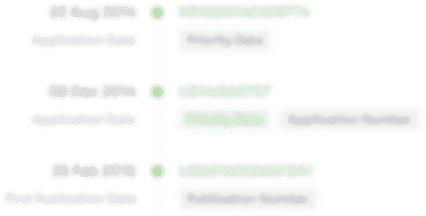
- R&D Engineer
- R&D Manager
- IP Professional
- Industry Leading Data Capabilities
- Powerful AI technology
- Patent DNA Extraction
Browse by: Latest US Patents, China's latest patents, Technical Efficacy Thesaurus, Application Domain, Technology Topic.
© 2024 PatSnap. All rights reserved.Legal|Privacy policy|Modern Slavery Act Transparency Statement|Sitemap