Running behavior recognition method based on convolutional neural network and acceleration sensor
A convolutional neural network and acceleration sensor technology, applied in the field of intelligent identification software, can solve problems such as inability to accurately identify replacement running, and achieve the effect of cost advantages, good cost advantages, and high accuracy.
- Summary
- Abstract
- Description
- Claims
- Application Information
AI Technical Summary
Problems solved by technology
Method used
Image
Examples
Embodiment 1
[0086] As a preferred embodiment of the present invention, refer to the accompanying image 3 As shown, this embodiment discloses a running behavior recognition method based on a convolutional neural network and an acceleration sensor, including the following steps:
[0087] S1. After the running starts, obtain the data of the three-axis acceleration sensor in the device carried by the user in real time;
[0088] S2. Sampling the data obtained in step S1 at a set time interval t; continuously collect sampling data in a time period T, and continuously collect sampling data in multiple time periods T, until the running ends;
[0089] S3. Perform fitting and resampling on the sampled data in multiple time periods T continuously collected in step S2 through cubic spline interpolation, and each time period T corresponds to a time series with a fixed length of L, and multiple For multiple time series corresponding to time period T, each time series is taken as a sample data to obta...
Embodiment 2
[0113] As another preferred embodiment of the present invention, refer to the accompanying figure 1 , attached figure 2 and attached image 3 As shown, this embodiment discloses a running behavior recognition method based on a convolutional neural network and an acceleration sensor. In this embodiment, the method is configured as a smart phone App, or a plug-in module running in various college running apps.
[0114] The data of the three-axis acceleration sensor in the smartphone can be acquired in real time. The data consists of a total of 6 features (the x, y, and z-axis components of the two acceleration vectors) including the gravitational acceleration and the two acceleration vectors that do not include the gravitational acceleration. By calculating the modulo length of these two vectors, 2 additional features are obtained and merged with 6 features, the resulting data has a total of 8 features.
[0115] Sampling at a time interval of 50ms, a time series composed of...
Embodiment 3
[0137] As another preferred embodiment of the present invention, this embodiment can be used as a specific application example to the above-mentioned Embodiment 1 and Embodiment 2. After the method is integrated into a mobile phone APP, the specific use process is as follows:
[0138] (1) Students open the APP software and start running and punching in;
[0139] (2) The software starts to collect the current three-axis acceleration data every 50ms, and calculates its modulo lengths respectively to obtain 8 features;
[0140] (3) When a total of 5s of data is collected, a time series data is obtained, and the time series is fitted by the cubic interpolation method and re-sampled to obtain time series data with a length of 64;
[0141] (4) Input the time series into the convolutional neural network SKSNet;
[0142] (5) Record the behavior detection results obtained by the model;
[0143] (6) If the student does not click to finish running, repeat steps (1) to (5);
[0144] (7...
PUM
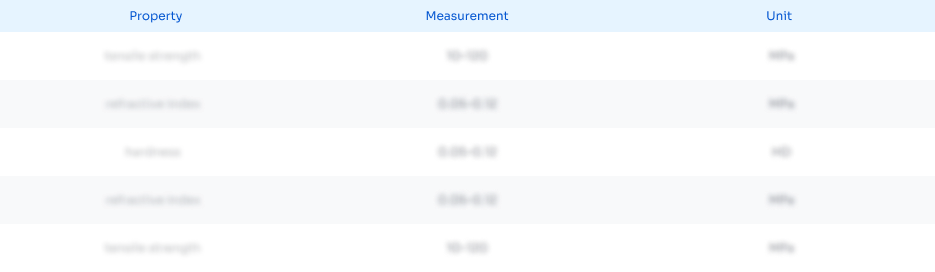
Abstract
Description
Claims
Application Information
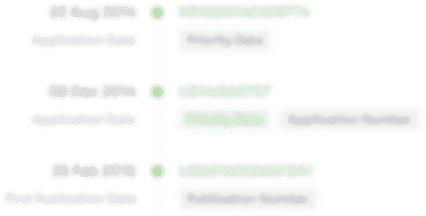
- R&D
- Intellectual Property
- Life Sciences
- Materials
- Tech Scout
- Unparalleled Data Quality
- Higher Quality Content
- 60% Fewer Hallucinations
Browse by: Latest US Patents, China's latest patents, Technical Efficacy Thesaurus, Application Domain, Technology Topic, Popular Technical Reports.
© 2025 PatSnap. All rights reserved.Legal|Privacy policy|Modern Slavery Act Transparency Statement|Sitemap|About US| Contact US: help@patsnap.com