Bad data identification method based on coupling of proximity analysis and neural network prediction
A technology of coupling neural network and bad data, which is applied in the field of bad data identification based on proximity analysis coupling neural network prediction, can solve the problems of difficulty in guaranteeing the accuracy of bad data identification, lack of mathematical statistical models, and heavy workload of manual judgment. , achieve powerful nonlinear fitting and generalization capabilities, clear theory, and prevent misjudgment
- Summary
- Abstract
- Description
- Claims
- Application Information
AI Technical Summary
Problems solved by technology
Method used
Image
Examples
Embodiment 1
[0036] A bad data identification method based on proximity analysis coupled neural network prediction, such as figure 1 The specific implementation steps are as follows:
[0037] Step 1. A total of 1000 groups of raw data samples U0 for accurate operation collected from the SCR denitration system, each group of samples includes inlet pressure, inlet flue gas volume, inlet NO x Concentration, ammonia injection amount, flue gas temperature, outlet pressure, outlet NO x A total of 10 parameters including concentration, denitration efficiency, ammonia escape concentration, and SCR denitration catalyst activity were selected. 50 groups of samples were randomly selected from the original data sample U0, and an error of 10% of the value was artificially added. Outline processing, recorded as sample set U1:
[0038]
[0039] In the formula, Z ni is the normalized value of parameter Z, Z i is the original value of parameter Z, Z max is the maximum value of parameter Z, Z min i...
Embodiment 2
[0051] A bad data identification method based on proximity analysis coupled neural network prediction, such as figure 1 The specific implementation steps are as follows:
[0052] Step 1. A total of 1000 groups of raw data samples U0 for accurate operation collected from the SCR denitration system, each group of samples includes inlet pressure, inlet flue gas volume, inlet NO x Concentration, ammonia injection amount, flue gas temperature, outlet pressure, outlet NO x A total of 10 parameters including concentration, denitration efficiency, ammonia escape concentration, and SCR denitration catalyst activity were selected. 50 groups of samples were randomly selected from the original data sample U0, and an error of 10% of the value was artificially added. Outline processing, recorded as sample set U1:
[0053]
[0054] In the formula, Z ni is the normalized value of parameter Z, Z i is the original value of parameter Z, Z max is the maximum value of parameter Z, Z min i...
PUM
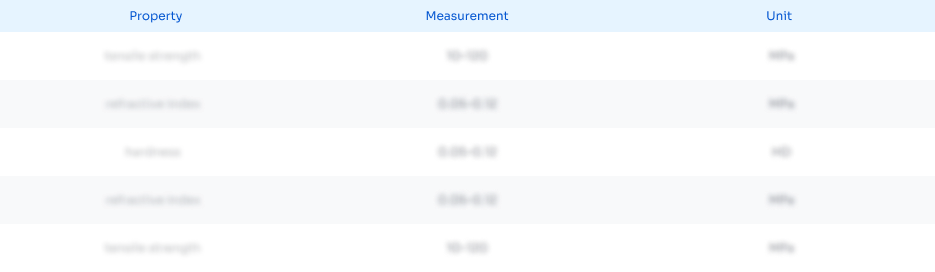
Abstract
Description
Claims
Application Information
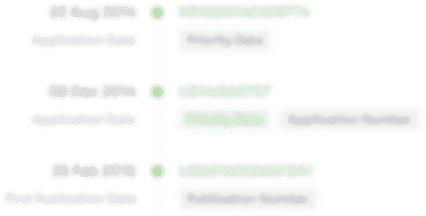
- Generate Ideas
- Intellectual Property
- Life Sciences
- Materials
- Tech Scout
- Unparalleled Data Quality
- Higher Quality Content
- 60% Fewer Hallucinations
Browse by: Latest US Patents, China's latest patents, Technical Efficacy Thesaurus, Application Domain, Technology Topic, Popular Technical Reports.
© 2025 PatSnap. All rights reserved.Legal|Privacy policy|Modern Slavery Act Transparency Statement|Sitemap|About US| Contact US: help@patsnap.com