Remote sensing image cultivated land parcel extraction method combining edge detection and multi-task learning
A multi-task learning and edge detection technology, applied in the field of remote sensing image analysis, can solve the problems of difficulty in obtaining continuous and closed plot boundaries, low extraction accuracy, etc., to achieve seamless splicing, improve geometric accuracy, and overcome the problem of splicing traces. Effect
- Summary
- Abstract
- Description
- Claims
- Application Information
AI Technical Summary
Problems solved by technology
Method used
Image
Examples
Embodiment 1
[0107] In this example,
[0108] In step S41, the root mean error is defined as follows:
[0109]
[0110] In the formula, L dist is the root mean loss, D p (x), D(x) are the distance value predicted by the model and the real distance value, respectively. Among them, x is the pixel position in the image space R, and N is the number of input samples, which is 2528.
[0111] In this embodiment, in step S41, the two-category cross-entropy loss is defined as follows:
[0112]
[0113] In the formula, l boun (W) is the total boundary loss, W represents all the learned parameters in the RCF model, is the activation value of the kth (k=5) level in the RCF model, |R| represents the number of pixels in the image R; for each boundary point, define a hyperparameter ε, set to 0.5: if a point is an edge If the probability of the point is greater than ε, the point is considered to be the boundary; if the probability of this point is 0, it is not the boundary; in addition, the p...
Embodiment 2
[0131] In this example, the GF-1 PMS image of the 21st Regiment of the Second Agricultural Division of Xinjiang in September 2018 is used, the spatial resolution after preprocessing is 2m, and the bands are red, green, and blue. This example uses 2528 images with a pixel size of 256×256 and the corresponding parcel labels for model training.
[0132] like figure 2 As shown, it is a structural diagram of the edge detection model RCF of this embodiment. As can be seen from the figure, RCF is an edge detection model with a VGG16 network backbone, and the model is completed by five layers. Each convolutional layer in the VGG16 network is followed by a convolutional operation with a channel number of 21, and a convolutional layer with a kernel size of 1×1. Next, use an upsampling operation (ie figure 2 Deconv layer in ) to restore the resolution of the feature maps, and then use the Sigmoid activation function to get the feature maps for each stage. Finally, a 1×1 convolution...
PUM
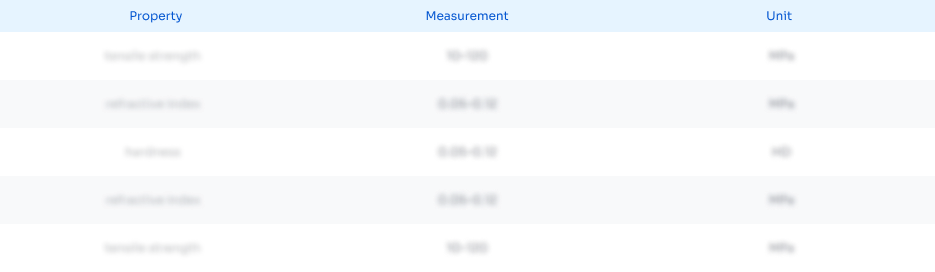
Abstract
Description
Claims
Application Information
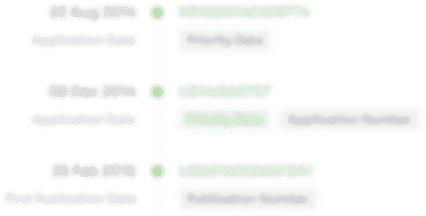
- Generate Ideas
- Intellectual Property
- Life Sciences
- Materials
- Tech Scout
- Unparalleled Data Quality
- Higher Quality Content
- 60% Fewer Hallucinations
Browse by: Latest US Patents, China's latest patents, Technical Efficacy Thesaurus, Application Domain, Technology Topic, Popular Technical Reports.
© 2025 PatSnap. All rights reserved.Legal|Privacy policy|Modern Slavery Act Transparency Statement|Sitemap|About US| Contact US: help@patsnap.com