Bearing fault detection method for unbalanced data SVM (support vector machine)
A technology for fault detection and equalization of data, which is applied to measuring devices, measuring ultrasonic/sonic/infrasonic waves, instruments, etc., can solve the problems of large randomness, easy loss of useful information, removal of redundant information and incomplete noise, etc., to achieve improvement Detection performance, strong removal effect
- Summary
- Abstract
- Description
- Claims
- Application Information
AI Technical Summary
Problems solved by technology
Method used
Image
Examples
Embodiment Construction
[0020] The present invention is described in more detail below in conjunction with accompanying drawing example:
[0021] combine Figure 1-6 , a kind of unbalanced data SVM bearing fault detection method of the present invention, comprises the following steps:
[0022] (1) Utilize the sensor installed on the output shaft of the induction motor to collect the vibration signal;
[0023] (2) Use the mutual information function index and the false nearest neighbor method to determine the embedding dimension and the delay time interval, and use the time delay technology to reconstruct the normal sample phase space, and the projection coefficient of the fault type sample in the normal sample space is the fault characteristic;
[0024] (3) α represents the number of normal samples that need to be deleted and the ratio of the difference between the number of normal samples and the number of faulty samples. The initial value of α is 0.3, and the initial value of α is used to compare...
PUM
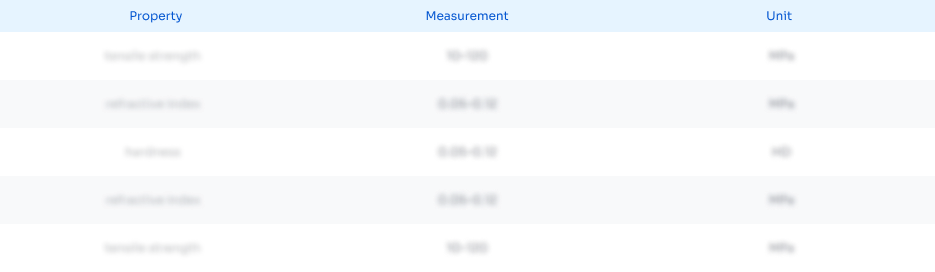
Abstract
Description
Claims
Application Information
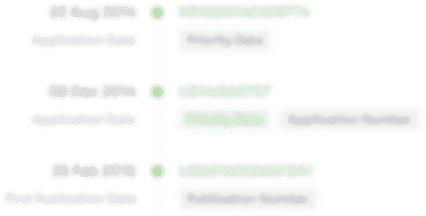
- R&D Engineer
- R&D Manager
- IP Professional
- Industry Leading Data Capabilities
- Powerful AI technology
- Patent DNA Extraction
Browse by: Latest US Patents, China's latest patents, Technical Efficacy Thesaurus, Application Domain, Technology Topic, Popular Technical Reports.
© 2024 PatSnap. All rights reserved.Legal|Privacy policy|Modern Slavery Act Transparency Statement|Sitemap|About US| Contact US: help@patsnap.com