Bidirectional clustering detection method for local similarity submatrices in data matrix
A technology of similarity sub-matrix and data matrix, applied in structured data retrieval, database model, relational database, etc., can solve problems, increase the difficulty of distinguishing, limited types of local similarity sub-matrix, etc., and achieve reduced labor intensity and good results readability effect
- Summary
- Abstract
- Description
- Claims
- Application Information
AI Technical Summary
Problems solved by technology
Method used
Image
Examples
Embodiment Construction
[0012] The present invention proposes a new two-way clustering detection technology framework system, designs and realizes a complete local similarity sub-matrix detection process based on clustering results, and combines traditional clustering algorithms (such as K-means, FCM, etc.) with local similarity sub-matrix The matrix detection is separated into two independent sequential processes as attached figure 1 As shown, the detection of the local similarity sub-matrix is based on the clustering results of traditional clustering algorithms. The following is attached figure 2 The specific detection steps of the present invention are described in detail:
[0013] (1) Preprocessing of the original data matrix: Suppose the original data matrix D consists of m rows and n columns, as attached figure 2 As shown, first judge the size of the number of row numbers m and the number of column numbers n, if n≥m, transpose the data matrix D to obtain a new data matrix D consisting of n row...
PUM
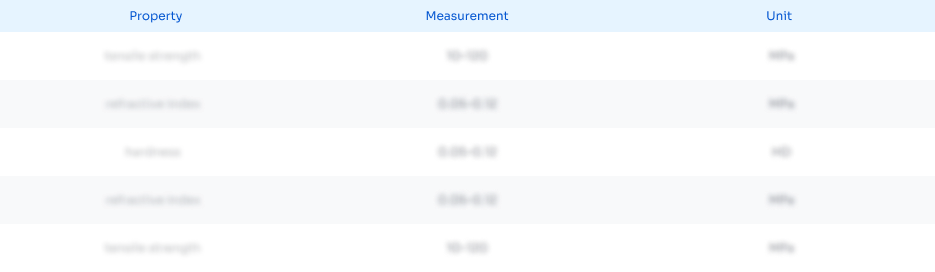
Abstract
Description
Claims
Application Information
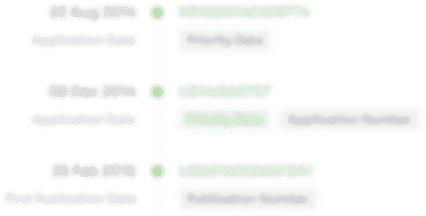
- R&D Engineer
- R&D Manager
- IP Professional
- Industry Leading Data Capabilities
- Powerful AI technology
- Patent DNA Extraction
Browse by: Latest US Patents, China's latest patents, Technical Efficacy Thesaurus, Application Domain, Technology Topic, Popular Technical Reports.
© 2024 PatSnap. All rights reserved.Legal|Privacy policy|Modern Slavery Act Transparency Statement|Sitemap|About US| Contact US: help@patsnap.com