Internet of Things data uncertainty measurement, prediction and outlier-removing method based on Gaussian process
A Gaussian process and uncertainty technology, which is used in the quality control of the automatic observation data of the Internet of Things, the uncertainty measurement of the Internet of Things data based on the Gaussian process, the field of prediction and outlier elimination, and can solve the problem of low accuracy and large training data. , the slow convergence speed of the neural network method, etc., to achieve the effect of simple and feasible method, high accuracy and small prediction error
- Summary
- Abstract
- Description
- Claims
- Application Information
AI Technical Summary
Problems solved by technology
Method used
Image
Examples
Embodiment Construction
[0032] Below in conjunction with accompanying drawing, the patent of the present invention is further described.
[0033] This method adopts the Gaussian process modeling theory and the dynamic system method of autoregressive model characterization to carry out the specific steps of uncertainty measurement, missing value prediction and outlier elimination for the time series data collected by the Internet of Things (such as figure 1 )as follows:
[0034] (1) Collect the standard deviation of the measurement error of the IoT sensor
[0035] According to the range range of the Internet of Things sensing sensor, the calibration test plan is formulated, the standard physical quantity is measured by the Internet of Things sensing sensor, the error of the measurement data transmitted by the sensor perception, the Internet of Things, and the wide area communication network is counted, and the detected quantity in the calibration test plan is calculated. The standard deviation σ of t...
PUM
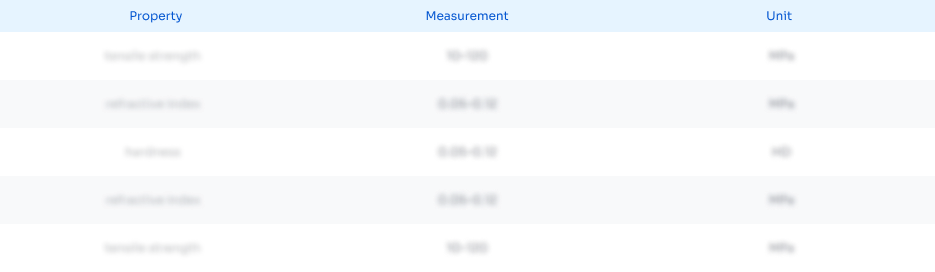
Abstract
Description
Claims
Application Information
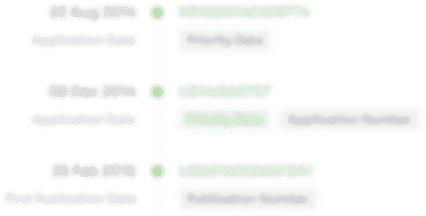
- R&D Engineer
- R&D Manager
- IP Professional
- Industry Leading Data Capabilities
- Powerful AI technology
- Patent DNA Extraction
Browse by: Latest US Patents, China's latest patents, Technical Efficacy Thesaurus, Application Domain, Technology Topic, Popular Technical Reports.
© 2024 PatSnap. All rights reserved.Legal|Privacy policy|Modern Slavery Act Transparency Statement|Sitemap|About US| Contact US: help@patsnap.com