Feature gene selection method based on logistic and relevant information entropy
A technology of characteristic genes and related information, applied in the field of data processing, can solve problems such as poor generalization performance and high risk of overfitting, and achieve the effects of reducing workload, optimizing data quality, and avoiding information loss
- Summary
- Abstract
- Description
- Claims
- Application Information
AI Technical Summary
Problems solved by technology
Method used
Image
Examples
example
[0114] In this paper, the breast cancer data set (Breast) and the gastric cancer data set (Bastric) in the UCI database are used as experimental data, and the classifier LIBSVM is used to select the parameters and features at the same time to find the optimal point of the corresponding parameters. The kernel function is RBF. Since the genetic data sample is small, the value of the penalty factor can be increased. If the value is larger, it indicates that the emphasis on each sample is stronger. Therefore, the penalty factor c=100 in this paper, and other parameters are default. Table 1 is the description of the experimental data, and Table 2 is the classification performance comparison of the three algorithms.
[0115] Table 1 Description of experimental data set
[0116]
[0117]
[0118] Table 2 Classification performance comparison of three algorithms
[0119]
PUM
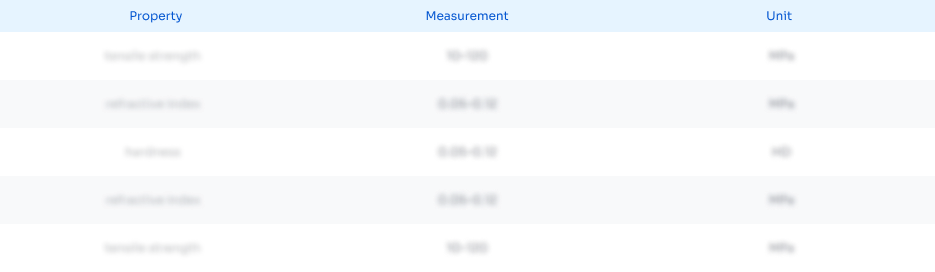
Abstract
Description
Claims
Application Information
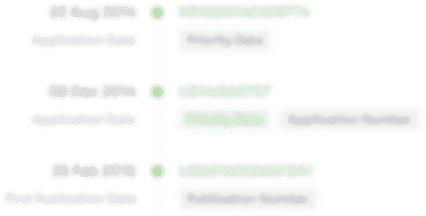
- R&D Engineer
- R&D Manager
- IP Professional
- Industry Leading Data Capabilities
- Powerful AI technology
- Patent DNA Extraction
Browse by: Latest US Patents, China's latest patents, Technical Efficacy Thesaurus, Application Domain, Technology Topic, Popular Technical Reports.
© 2024 PatSnap. All rights reserved.Legal|Privacy policy|Modern Slavery Act Transparency Statement|Sitemap|About US| Contact US: help@patsnap.com