Deep-learning-based pedestrian re-identification method
A deep learning and re-identification technology, applied in the field of pedestrian re-identification, can solve the problems of feature expressiveness and discriminative limitation, and the reduction of matching effect
- Summary
- Abstract
- Description
- Claims
- Application Information
AI Technical Summary
Problems solved by technology
Method used
Image
Examples
Embodiment
[0036] Such as figure 1 As shown, the pedestrian re-identification method based on deep convolutional neural network of the present invention comprises the following steps:
[0037] S1. Propose a deep network structure suitable for pedestrian re-identification. The deep network structure adopts an eight-layer structure, including five convolutional layers and three fully connected layers. The deep network takes a pair of pedestrian images as input, directly Their similarity scores are obtained from the raw pixels of the original image. It does not require any preprocessing of the original image, nor does it need to manually design complex features and descriptors.
[0038] Such as figure 2 As shown, the convolution kernels of the first five convolutional layers can be regarded as a plurality of local feature detectors, starting from the bare pixels of the input image, extracting robustness to illumination, attitude, camera angle changes, etc. feature. The fully connected ...
PUM
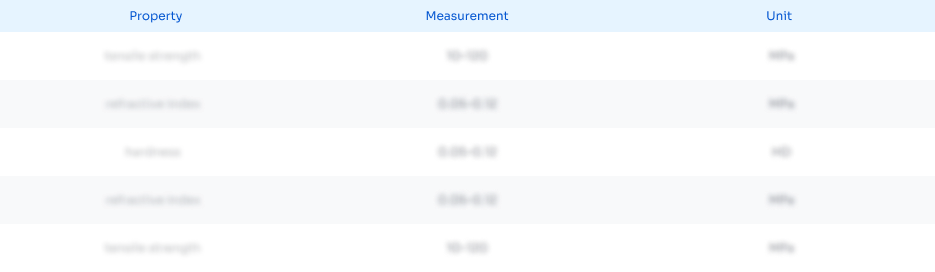
Abstract
Description
Claims
Application Information
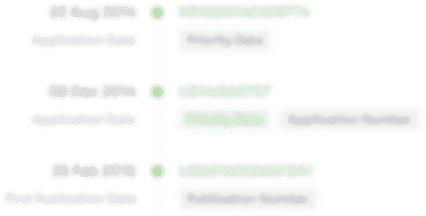
- R&D Engineer
- R&D Manager
- IP Professional
- Industry Leading Data Capabilities
- Powerful AI technology
- Patent DNA Extraction
Browse by: Latest US Patents, China's latest patents, Technical Efficacy Thesaurus, Application Domain, Technology Topic, Popular Technical Reports.
© 2024 PatSnap. All rights reserved.Legal|Privacy policy|Modern Slavery Act Transparency Statement|Sitemap|About US| Contact US: help@patsnap.com