Modeling method for multi-scale forecasting of ultra-short-term wind speed based on generative deep confidence network
A technology of deep belief network and modeling method, which is applied in the field of ultra-short-term wind speed multi-scale forecasting and modeling based on generative deep belief network, which can solve the problems of limited forecasting accuracy and inability to deal with wavelet decomposition of high-frequency components. , to achieve the effects of easy multi-step forecasting, multi-step forecasting, and easy adjustment
- Summary
- Abstract
- Description
- Claims
- Application Information
AI Technical Summary
Problems solved by technology
Method used
Image
Examples
specific Embodiment approach 1
[0021] The ultra-short-term wind speed multi-scale forecast modeling method based on the generative deep belief network includes the following steps:
[0022] Step 1. Record the wind speed time series (X 1 ,X 2 ,...,X k ,X k+1 ,...,X k+l ) is a sample point set with a length of k+l; where (X 1 ,X 2 ,...,X k ) as training sample points, the length is k, (X k+1 ,X k+2 ...,X k+l ) as a test sample point, the length is l; use t layer wavelet decomposition to process wind speed data, and obtain t+1 segments of different frequencies c t , d 1 , d 2 ,... d t The wind speed sequence whose length is k+l; c t Indicates the low frequency component, d 1 , d 2 ,... d t Indicates high-frequency components;
[0023] Step 2. Build training set and test set:
[0024] Construct the low frequency component c t The following training set and test set: low frequency component c t The sequence is denoted as (x 1 ,x 2 ,...,x k ,x k+1 ,...,x k+l ), will (x 1 ,x 2 ,...,x k ...
specific Embodiment approach 2
[0028] The wavelet decomposition described in Step 1 of this embodiment is realized by using the Mallat algorithm.
[0029]Other steps and parameters are the same as those in Embodiment 1.
specific Embodiment approach 3
[0030] The specific process of using t-layer wavelet decomposition to process wind speed data described in step 1 of the present embodiment is as follows:
[0031] like figure 2 As shown, the time series (X 1 ,X 2 ,...,X k ,X k+1 ,...,X k+l ) is decomposed into low frequency components c 1 and the high frequency component d 1 ; When further decomposing the sequence, only the low frequency component c 1 decomposed into c 2 and d 2 , and do not decompose the high-frequency components, and decompose the decomposed low-frequency components in turn, and finally obtain the low-frequency component c t and the high frequency component d 1 , d 2 ,...,d t , so as to separate the trend item and the fluctuation item.
[0032] Other steps and parameters are the same as those in Embodiment 1 or Embodiment 2.
PUM
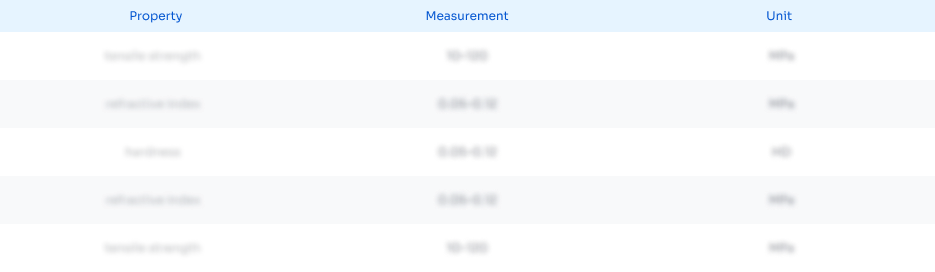
Abstract
Description
Claims
Application Information
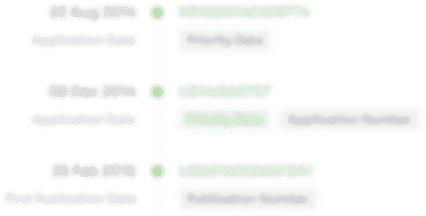
- R&D
- Intellectual Property
- Life Sciences
- Materials
- Tech Scout
- Unparalleled Data Quality
- Higher Quality Content
- 60% Fewer Hallucinations
Browse by: Latest US Patents, China's latest patents, Technical Efficacy Thesaurus, Application Domain, Technology Topic, Popular Technical Reports.
© 2025 PatSnap. All rights reserved.Legal|Privacy policy|Modern Slavery Act Transparency Statement|Sitemap|About US| Contact US: help@patsnap.com