Multimode system feature dimensionality reduction method
A feature dimension reduction and multi-mode technology, applied in character and pattern recognition, instruments, computer components, etc., can solve the problem of not being able to handle data equidistant manifolds well, without considering the similarity of low-dimensional space feature distances, data retention issues
- Summary
- Abstract
- Description
- Claims
- Application Information
AI Technical Summary
Problems solved by technology
Method used
Image
Examples
Embodiment Construction
[0032] like figure 1 As shown, a feature dimensionality reduction method for multi-modal systems, the method includes the following sequential steps:
[0033] (1) Acquisition of different working modes of the multi-mode system F n M groups of sample feature vectors under n=1,2,...,N, m=1,2,...,M, is a column vector, representing the mth sample feature vector of the nth type of pattern, N represents the total number of patterns of the system, and N>3, D represents the original dimension of the sample feature vector, and satisfies D>N-1;
[0034] (2) To sample feature vector in turn Perform standardization processing to obtain the standardized sample feature vector Its calculation method is: Where ||·|| represents the 2-norm of the vector;
[0035] (3) Constructing a standardized feature matrix for multimodal samples
[0036] The subscripts Z and D are used to indicate the dimension of the matrix, that is, the standardized feature matrix is a matrix of Z rows a...
PUM
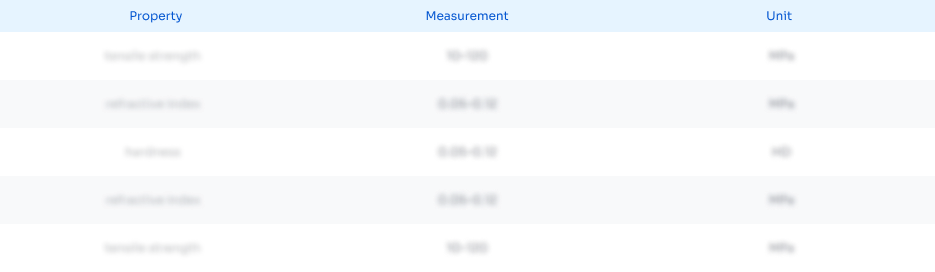
Abstract
Description
Claims
Application Information
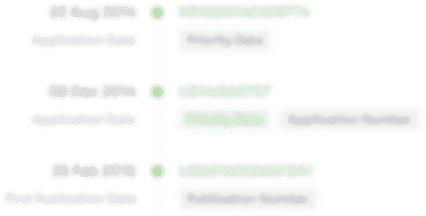
- R&D
- Intellectual Property
- Life Sciences
- Materials
- Tech Scout
- Unparalleled Data Quality
- Higher Quality Content
- 60% Fewer Hallucinations
Browse by: Latest US Patents, China's latest patents, Technical Efficacy Thesaurus, Application Domain, Technology Topic, Popular Technical Reports.
© 2025 PatSnap. All rights reserved.Legal|Privacy policy|Modern Slavery Act Transparency Statement|Sitemap|About US| Contact US: help@patsnap.com