Method and system for hemorrhage area segmentation in brain CT images based on semi-supervised learning
A semi-supervised learning and CT image technology, applied in image analysis, image enhancement, graphics and image conversion, etc., can solve the problems of ignoring inter-frame information and poor effect, and achieve the effect of simple processing method and easy extraction
- Summary
- Abstract
- Description
- Claims
- Application Information
AI Technical Summary
Problems solved by technology
Method used
Image
Examples
Embodiment Construction
[0061] The invention is applicable to the hemorrhage area segmentation in the medical cranial CT image, and is a method for segmenting the hemorrhage area of the brain CT image based on semi-supervised learning and three-dimensional supervoxel.
[0062] The flow chart of the present invention is as figure 1 , mainly including the Tri-training model training stage and the bleeding area segmentation stage based on the Tri-training model.
[0063] The Tri-training model training phase includes the following steps:
[0064] (1.1) Converting the CT image format: Obtain the CT image sequence containing the hemorrhage area from the computer tomography equipment or database, intercept the effective interval of the pixel value, and convert it into a commonly used computer image processing format. figure 2 That is, the image obtained after the format conversion of the CT image.
[0065] (1.2) Mark training samples: Divide the CT image sequence into two parts, one part of the sequen...
PUM
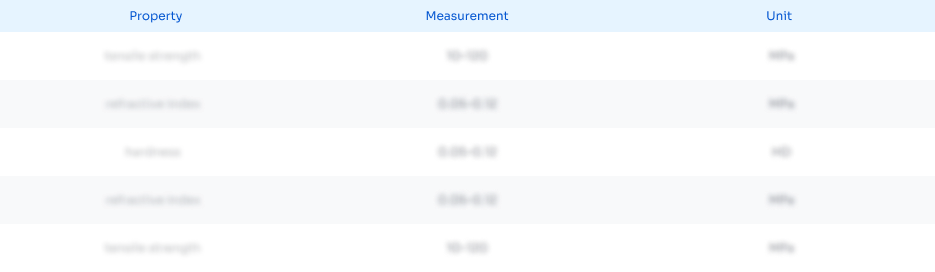
Abstract
Description
Claims
Application Information
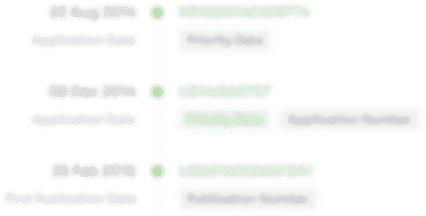
- R&D Engineer
- R&D Manager
- IP Professional
- Industry Leading Data Capabilities
- Powerful AI technology
- Patent DNA Extraction
Browse by: Latest US Patents, China's latest patents, Technical Efficacy Thesaurus, Application Domain, Technology Topic, Popular Technical Reports.
© 2024 PatSnap. All rights reserved.Legal|Privacy policy|Modern Slavery Act Transparency Statement|Sitemap|About US| Contact US: help@patsnap.com