Resource allocation method for reinforcement learning in ultra-dense network
An ultra-dense network and resource allocation technology, applied in network topology, wireless communication, power management, etc., can solve problems such as cross-layer interference in 5G networks that cannot be solved well
- Summary
- Abstract
- Description
- Claims
- Application Information
AI Technical Summary
Problems solved by technology
Method used
Image
Examples
Embodiment Construction
[0037] The main idea of the present invention is to detect the state of the current channel by simulating the communication environment, establishing a model, initializing the learning factor, conjecture, transmission strategy and evaluation function Q, and the state indication parameters include SIR, transmit power, and state Wait. Select the current action according to the transmission strategy, compare the detected signal-to-interference ratio with a given threshold, if it is greater than the threshold, get a reward, if it is smaller than the threshold, the reward is set to zero, and use the guess-based Q update formula to get a new According to the Q value, the strategy and guess of the next moment are obtained through the greedy strategy according to the Q value, the state of the next moment is updated, and the next communication state is entered, and the above learning process is repeated. The power allocation scheme is evaluated with the Q value as the performance eva...
PUM
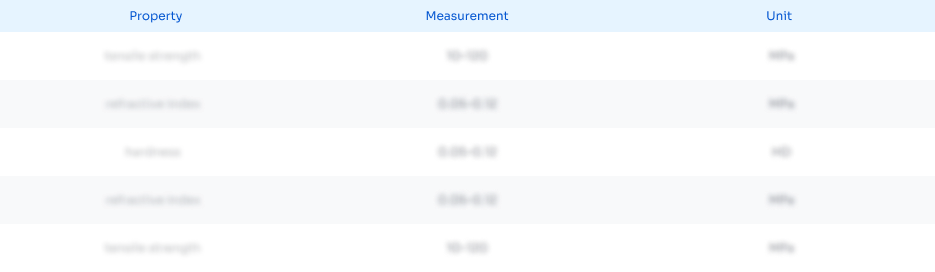
Abstract
Description
Claims
Application Information
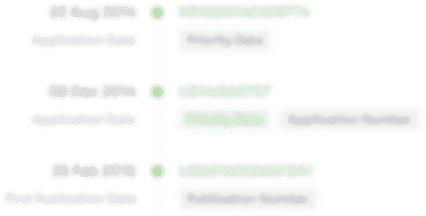
- R&D
- Intellectual Property
- Life Sciences
- Materials
- Tech Scout
- Unparalleled Data Quality
- Higher Quality Content
- 60% Fewer Hallucinations
Browse by: Latest US Patents, China's latest patents, Technical Efficacy Thesaurus, Application Domain, Technology Topic, Popular Technical Reports.
© 2025 PatSnap. All rights reserved.Legal|Privacy policy|Modern Slavery Act Transparency Statement|Sitemap|About US| Contact US: help@patsnap.com