Roadside air pollutant concentration prediction method based on reconstruction deep learning
An air pollutant and deep learning technology, applied in the field of roadside air pollutant concentration prediction based on reconstruction deep learning, can solve problems such as low feasibility, real-time and migration defects, and low model accuracy of complex roads , to achieve good migration effect
- Summary
- Abstract
- Description
- Claims
- Application Information
AI Technical Summary
Problems solved by technology
Method used
Image
Examples
Embodiment Construction
[0044] Such as figure 1 As shown, the specific implementation of the present invention is as follows:
[0045] 1. Based on the diversity of the inducing factors of the air pollutant concentration on the roadside and the correlation characteristics of historical data, combined with the characteristics of the restricted Boltzmann machine and the Elman network, construct a feedforward connection and feedback connection structure with local memory capabilities , The main network is composed of an input layer, a receiving layer, an intermediate layer, and an output layer. The secondary network used for the initialization of the main network contains a visible layer and a hidden layer. The number of input layer, output layer, and visible layer units are respectively 14, 3, 14 deep reconstruction of Elman model.
[0046] Such as figure 2 As shown, the left side of the figure is the secondary network, the right side of the figure is the main network, N is the number of visible units of t...
PUM
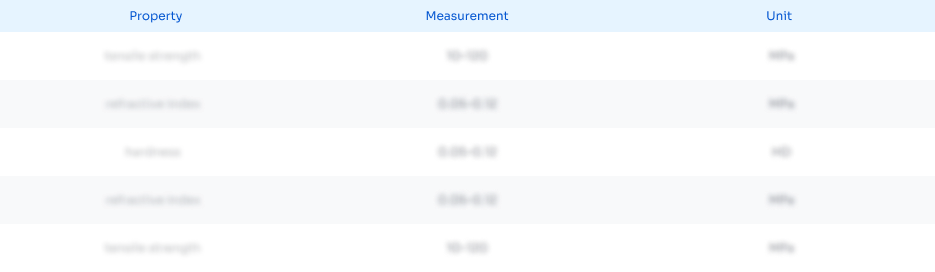
Abstract
Description
Claims
Application Information
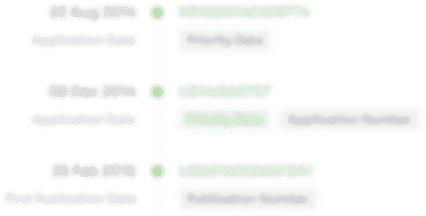
- R&D Engineer
- R&D Manager
- IP Professional
- Industry Leading Data Capabilities
- Powerful AI technology
- Patent DNA Extraction
Browse by: Latest US Patents, China's latest patents, Technical Efficacy Thesaurus, Application Domain, Technology Topic, Popular Technical Reports.
© 2024 PatSnap. All rights reserved.Legal|Privacy policy|Modern Slavery Act Transparency Statement|Sitemap|About US| Contact US: help@patsnap.com