Active Machine Learning System for Dangerous Host Monitoring
A machine learning and active learning technology, applied in the transmission system, electrical components, etc., can solve the problems of missing and processing important alarm information, high false alarm rate, and large alarm volume
- Summary
- Abstract
- Description
- Claims
- Application Information
AI Technical Summary
Problems solved by technology
Method used
Image
Examples
Embodiment
[0064] An active machine learning system for risky host monitoring that leverages SIEM alert information, various security logs, and analyst investigative notes to flag hosts with a high likelihood of compromise. The system includes data collection, feature engineering, label generation, machine learning, feedback algorithms for active learning analyst insights, and real-time alerting. The six parts are connected sequentially. After the data collection part collects and integrates various security-related information in the enterprise network, it is passed to the feature engineering part, and the feature engineering part standardizes the data and extracts features. After processing, the extracted feature vector is passed to the machine learning part as input data, and the label is generated by analyzing and mining the security analyst's investigation notes, extracting the security status label of the host, and sending it to the machine learning part as Learning objectives, the...
PUM
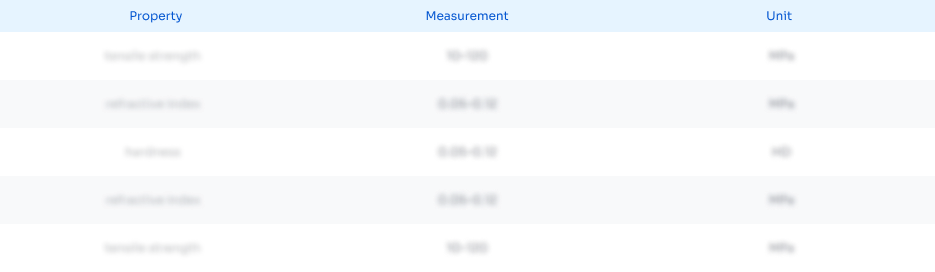
Abstract
Description
Claims
Application Information
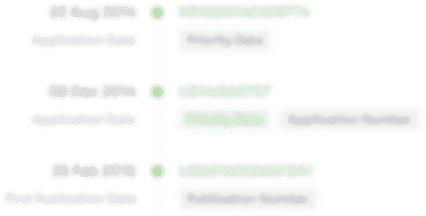
- R&D Engineer
- R&D Manager
- IP Professional
- Industry Leading Data Capabilities
- Powerful AI technology
- Patent DNA Extraction
Browse by: Latest US Patents, China's latest patents, Technical Efficacy Thesaurus, Application Domain, Technology Topic.
© 2024 PatSnap. All rights reserved.Legal|Privacy policy|Modern Slavery Act Transparency Statement|Sitemap