Image super-resolution reconstruction method of improved convolution network based on data enhancement
A super-resolution reconstruction and convolutional network technology, applied in the field of image super-resolution reconstruction, can solve the problems of no image information combined with the network structure, the reconstruction accuracy needs to be improved, and the network training convergence is slow, so as to avoid image resolution. The effect of descending, speeding up training convergence, and restoring image resolution
- Summary
- Abstract
- Description
- Claims
- Application Information
AI Technical Summary
Problems solved by technology
Method used
Image
Examples
Embodiment
[0022] The present invention is based on the image super-resolution reconstruction method of the improved convolution network of data enhancement, and concrete steps are as follows:
[0023] (1) Data enhancement: 91 images in the sample set are rotated 90°, 180°, 270°, flipped 0°, 90°, 180°, 270° seven operations, and then the steps are r=14, with overlap The cropping of 168000 fsub×fsub sub-images is obtained as the original HR image, where fsub=33; then Gaussian blur downsampling is performed on the original image to obtain the input dataset {Yi}.
[0024] (2) Network structure improvement: Construct a four-layer network model, such as figure 1 As shown, the first three layers of the network are composed of convolutional layers, which are used to extract image feature information as a feature extraction layer; the last layer is a deconvolutional layer as a reconstruction layer, and the feature information obtained by the convolutional layer is used for reconstruction, thus o...
PUM
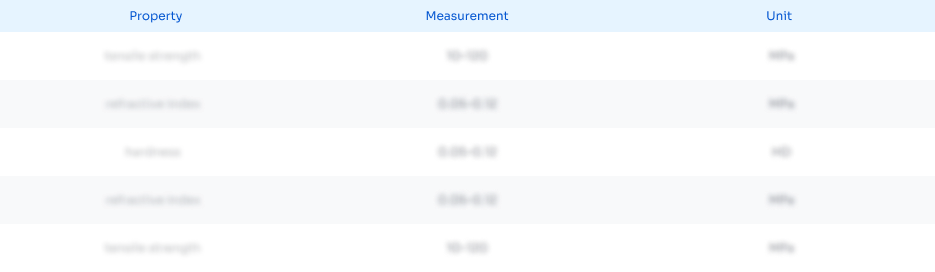
Abstract
Description
Claims
Application Information
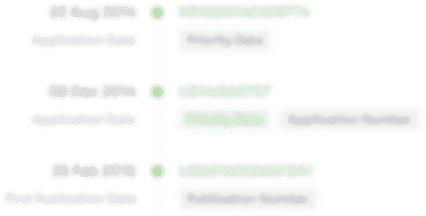
- R&D Engineer
- R&D Manager
- IP Professional
- Industry Leading Data Capabilities
- Powerful AI technology
- Patent DNA Extraction
Browse by: Latest US Patents, China's latest patents, Technical Efficacy Thesaurus, Application Domain, Technology Topic.
© 2024 PatSnap. All rights reserved.Legal|Privacy policy|Modern Slavery Act Transparency Statement|Sitemap