SAR image change detection system and method based on sparse auto-encoder and convolution neural network
A technology of convolutional neural network and sparse autoencoder, which is applied in biological neural network models, image enhancement, image analysis, etc., can solve problems such as being easily affected by speckle noise, failing to meet requirements, and affecting detection accuracy, and achieving The effects of improving accuracy, correct detection rate, and Kappa coefficient
- Summary
- Abstract
- Description
- Claims
- Application Information
AI Technical Summary
Problems solved by technology
Method used
Image
Examples
Embodiment Construction
[0041] The present invention will be further described in detail below in conjunction with specific embodiments, which are explanations of the present invention rather than limitations.
[0042] A SAR image change detection method based on a sparse autoencoder and a convolutional neural network in the present invention uses the sparse autoencoder SAE and the convolutional neural network CNN to perform feature extraction on the difference map DI, and converts the difference map DI into a feature space , reducing the impact of noise. At the same time, the present invention makes full use of the feature information of image pixels and the neighborhood information between pixels to greatly improve the accuracy rate of SAR image change detection. The realization process is as follows: (1) Input two registered SAR images of the same area at different times. (2) Calculate the two input images to obtain the difference map. (3) Use the sparse autoencoder SAE to extract features from ...
PUM
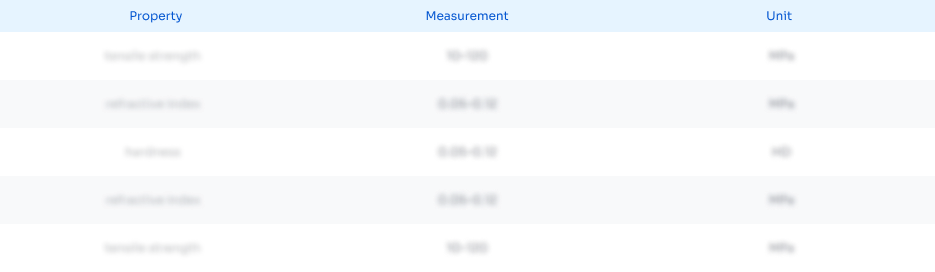
Abstract
Description
Claims
Application Information
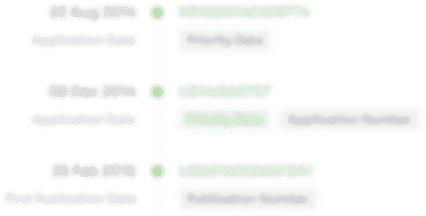
- R&D Engineer
- R&D Manager
- IP Professional
- Industry Leading Data Capabilities
- Powerful AI technology
- Patent DNA Extraction
Browse by: Latest US Patents, China's latest patents, Technical Efficacy Thesaurus, Application Domain, Technology Topic, Popular Technical Reports.
© 2024 PatSnap. All rights reserved.Legal|Privacy policy|Modern Slavery Act Transparency Statement|Sitemap|About US| Contact US: help@patsnap.com