Cow face identification method based on convolutional neural network and classifier model
A convolutional neural network and recognition method technology, applied in the field of computer vision and intelligent recognition, can solve the problems of cluttered background of cattle pictures, limited recognition range, and increased probability of wrong matching, achieve good anti-interference ability, reduce calculation The effect of time and recognition rate reduction
- Summary
- Abstract
- Description
- Claims
- Application Information
AI Technical Summary
Problems solved by technology
Method used
Image
Examples
Embodiment 1
[0065] This embodiment recognizes the cow face based on the convolutional neural network and the sparse representation classification model, such as figure 1 As shown in the flow chart of the cow face recognition method, the specific operation is carried out as follows.
[0066] 1. Collect cow face data, generate training data set and test data set
[0067] Such as figure 1 As shown in SE01, install a Yunshian H3-X color CMOS camera in front of the drinking fountain so that the camera sensor is basically parallel to the face of the cow drinking water standing in front of the drinking fountain, and adjust the camera position so that the field of view is 3 to 4 The width of the bull's face, and the height of the field of view is 1.2 to 1.5 bull's face length. Obtain video data of 30 cows drinking water during 8:00-17:00 on a fog-free and haze-free sunny day. The camera collects data 24 hours a day. The collected video is in PAL format and stored on a DS-7816N-K2 hard disk Ins...
Embodiment 2
[0124] On the basis of embodiment 1, the identification of the newly-added cattle is realized, specifically according to the following steps:
[0125] SN1. New cow face data collection: collect the cow face image of the new cow according to the method of data collection in step S1 (the new cow may be a newborn calf or a newly purchased cow from the farm), Unify the image size to the same size as the registered cattle image to form a new cattle data set;
[0126] SN2. Extract the image features of the newly added cow’s face: input the newly added cow’s face data into the CNN model initialized with the parameter λ, and extract the features of the 64-dimensional feature extraction layer of the CNN model as the image of the newly added cow. Cow face image feature data;
[0127] SN3. Newly added cattle registration: add the cow face image features of the newly added cows to the sparse representation classification model dictionary D obtained in step 4 of embodiment 1, specifically...
PUM
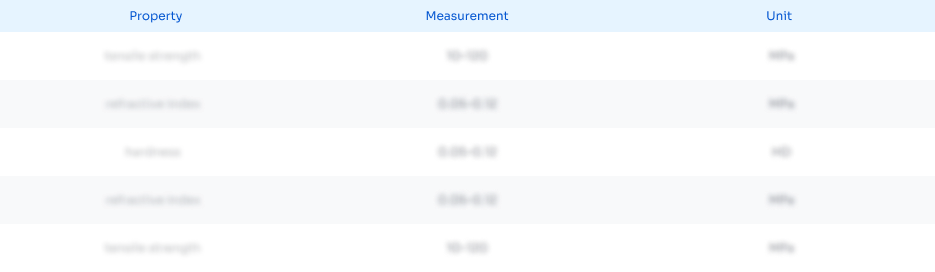
Abstract
Description
Claims
Application Information
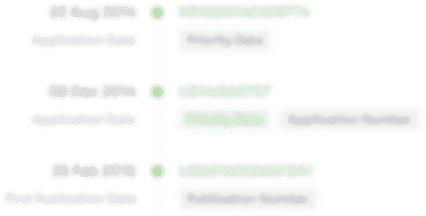
- R&D
- Intellectual Property
- Life Sciences
- Materials
- Tech Scout
- Unparalleled Data Quality
- Higher Quality Content
- 60% Fewer Hallucinations
Browse by: Latest US Patents, China's latest patents, Technical Efficacy Thesaurus, Application Domain, Technology Topic, Popular Technical Reports.
© 2025 PatSnap. All rights reserved.Legal|Privacy policy|Modern Slavery Act Transparency Statement|Sitemap|About US| Contact US: help@patsnap.com