High spectral image classification method based on NSCT transformation and DCNN
A technology of hyperspectral image and classification method, applied in the field of hyperspectral image classification based on non-subsampling contourlet transform and deep convolutional neural network, and the field of hyperspectral image classification, can solve the problem of compressing the spatial resolution of hyperspectral images, which cannot be effectively Extracting hyperspectral image detail information, hyperspectral image edge and texture detail information mining and other issues to improve classification performance and performance
- Summary
- Abstract
- Description
- Claims
- Application Information
AI Technical Summary
Problems solved by technology
Method used
Image
Examples
Embodiment 1
[0028] Hyperspectral remote sensing is a technology that uses imaging spectrometers to simultaneously image the ground surface with a band width of less than 10nm from the ultraviolet to the thermal infrared band of the electromagnetic spectrum. It can not only reflect the surface characteristics of the object, but also reflect the internal characteristics of the object. Therefore, it has been widely used in the field of remote sensing. Hyperspectral image classification is a very important field in the application of hyperspectral remote sensing, and it is also the basis of many other applications of hyperspectral remote sensing, so it is currently the most extensive field of hyperspectral remote sensing research.
[0029] At present, most of the classification methods of hyperspectral images are carried out in the spatial domain. While the spectral resolution of hyperspectral images is improved, the spatial resolution of hyperspectral images will inevitably be compressed, result...
Embodiment 2
[0041] The hyperspectral image classification method based on NSCT transform and DCNN is the same as that in Embodiment 1. The non-downsampled contourlet (NSCT) transform in step (2) of the present invention is performed according to the following steps:
[0042] (2a) Principal component analysis (PCA) is used to perform dimensionality reduction operations on the original hyperspectral image in the spectral direction, combining the energy retention of the principal components and the computational complexity of the subsequent NSCT transformation, the present invention retains the first three principal components.
[0043] (2b) Perform 3-level non-downsampled contourlet (NSCT) transformation on the three principal components obtained after dimensionality reduction. The sub-band coefficient matrix obtained by the transformation has an exponential relationship with the number of transformation stages, that is, the k-th transformation The low-frequency subband coefficient matrix obtaine...
Embodiment 3
[0048] The hyperspectral image classification method based on NSCT transform and DCNN is the same as that of embodiment 1-2. The block fetching operation described in step (4) of the present invention is performed according to the following steps:
[0049] (4a) In order to classify each pixel in the original hyperspectral image, before fetching the block, perform 2-layer 0 filling operation on the periphery of the stereo block obtained by superimposing the high-frequency subband coefficient matrix to obtain a size of (h+4 )×(w+4)×c three-dimensional block;
[0050] (4b) Taking each pixel as the center, use a 5×5 sliding window to fetch the three-dimensional block, and the size of the fetched block is 5×5×c;
[0051] (4c) Remove the block with the category label value of the center pixel point of 0, complete the block fetching operation, and obtain the sample set.
[0052] Because the spatial resolution of hyperspectral images is generally low, it is impossible to directly classify the...
PUM
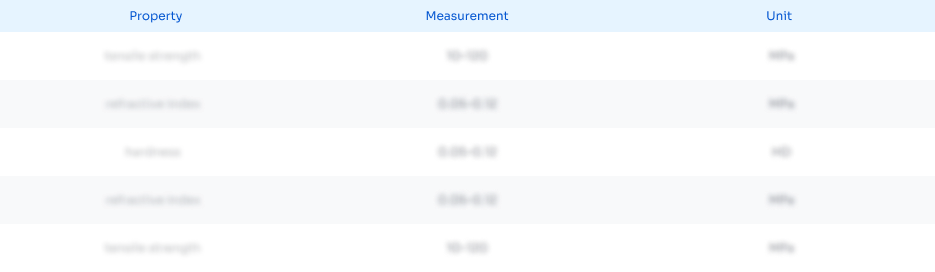
Abstract
Description
Claims
Application Information
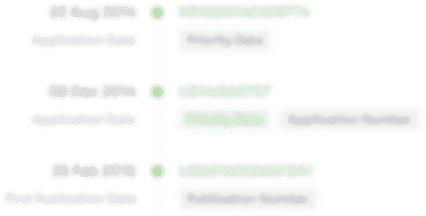
- R&D Engineer
- R&D Manager
- IP Professional
- Industry Leading Data Capabilities
- Powerful AI technology
- Patent DNA Extraction
Browse by: Latest US Patents, China's latest patents, Technical Efficacy Thesaurus, Application Domain, Technology Topic, Popular Technical Reports.
© 2024 PatSnap. All rights reserved.Legal|Privacy policy|Modern Slavery Act Transparency Statement|Sitemap|About US| Contact US: help@patsnap.com