SAR image change detection method based on stack semi-supervised adaptive denoising auto-encoder
An image change detection and self-encoder technology, applied in the field of image processing, can solve the problems of large influence of learning samples, errors, and inaccurate detection results, etc.
- Summary
- Abstract
- Description
- Claims
- Application Information
AI Technical Summary
Problems solved by technology
Method used
Image
Examples
Embodiment Construction
[0121] see figure 1 , the present invention provides a kind of SAR image change detection method based on unsupervised depth neural network, specifically comprises the following steps:
[0122] Step 1: Input phase 1 image I and phase 2 image J, I={I(u,v)|1≤u≤U,1≤v≤V}, J={J(u,v)|1 ≤u≤U,1≤v≤V}, where I(u,v) and J(u,v) are the gray values of image I and image J at pixel (u,v) respectively, where u and v They are the row number and column number of the image respectively, the maximum row number is U, and the maximum column number is V.
[0123] Step 2: Compute the Multiscale Difference Guidance Map
[0124] (2a) For the 3×3 neighborhood of the pixel at the position (u,v) in the phase 1 image I and the phase 2 image J, calculate the mean value of the 9 pixel values in the 3×3 neighborhood respectively, record for μ N3 (I(u,v)) and μ N3 (J(u,v)), and then calculate the 3×3 neighborhood mean difference value I at (u,v) according to the following formula S (u, v),
[0125] ...
PUM
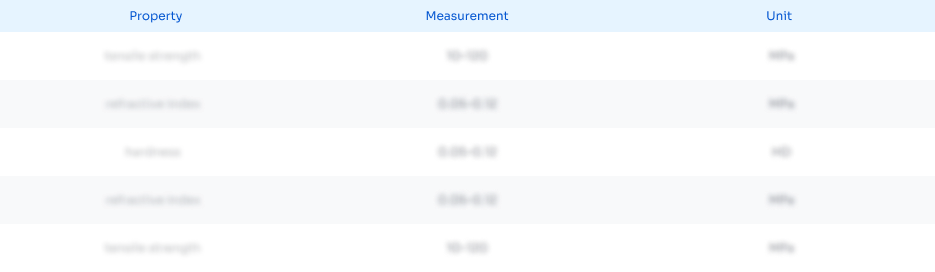
Abstract
Description
Claims
Application Information
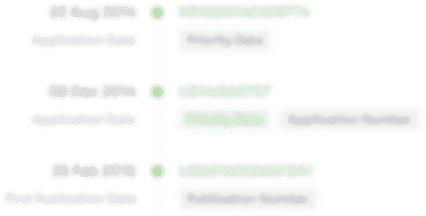
- R&D Engineer
- R&D Manager
- IP Professional
- Industry Leading Data Capabilities
- Powerful AI technology
- Patent DNA Extraction
Browse by: Latest US Patents, China's latest patents, Technical Efficacy Thesaurus, Application Domain, Technology Topic.
© 2024 PatSnap. All rights reserved.Legal|Privacy policy|Modern Slavery Act Transparency Statement|Sitemap