Discriminative association maximization hash-based cross-mode retrieval method
A cross-modal, discriminative technology, applied in character and pattern recognition, special data processing applications, instruments, etc., can solve the problem of not taking into account the discriminative distribution of data features, and achieve time reduction, easy classification, and improved performance. Effect
- Summary
- Abstract
- Description
- Claims
- Application Information
AI Technical Summary
Problems solved by technology
Method used
Image
Examples
Embodiment 1
[0068] This embodiment provides a cross-modal retrieval method based on discriminative association maximization hash, such as image 3 shown, including the following steps:
[0069] Step 1: Obtain a training data set, where each sample includes paired image and text modal data;
[0070] Step 2: Multimodal extraction is performed on the training data set to obtain the training multimodal data set O train ;
[0071] Step 3: For training multimodal dataset O train , to construct the objective function based on the discriminative association maximization hash on the data set;
[0072] Step 4: Solve the objective function, and obtain the projection matrix W of the projection of images and texts to the common Hamming space 1 and W 2 , the joint hash code B of the image-text pair and the classifier matrix Q, using the joint hash code B as the hash code of the pair of images and text;
[0073] Step 5: Obtain the test data set and perform multimodal extraction on it to obtain the...
Embodiment 2
[0129] According to the above cross-modal retrieval method based on discriminative correlation maximization hash, this embodiment provides a corresponding objective function construction method, such as figure 2 shown, including:
[0130] Step 1: Obtain a training data set, wherein each sample includes two modal data of paired images and text; multimodal extraction is performed on the training data set to obtain a training multimodal data set O train ;
[0131] Step 2: Project the data of the two modalities from the original heterogeneous space into the common Hamming space and maximize the association between pairs of images and texts in one sample;
[0132] Step 3: Perform linear discriminant analysis processing on the text modality data and transfer its characteristics to the image modality data;
[0133] Step 4: Convert the two modal data features into a hash code, and minimize the quantization loss of the hash code obtained through the hash function;
[0134] Step 5: ...
PUM
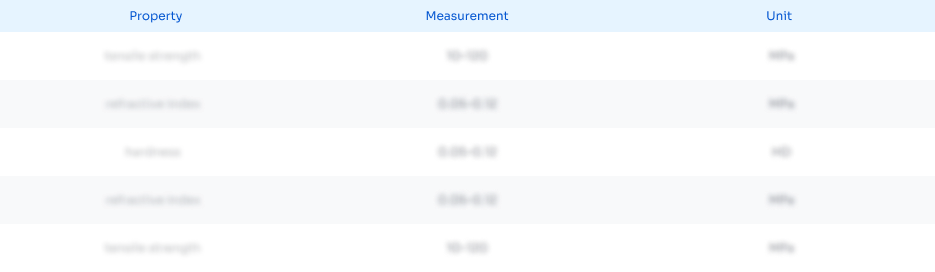
Abstract
Description
Claims
Application Information
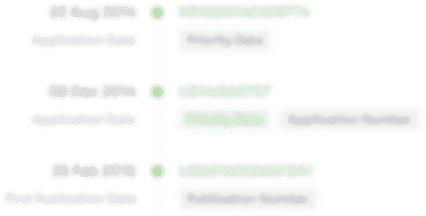
- R&D Engineer
- R&D Manager
- IP Professional
- Industry Leading Data Capabilities
- Powerful AI technology
- Patent DNA Extraction
Browse by: Latest US Patents, China's latest patents, Technical Efficacy Thesaurus, Application Domain, Technology Topic, Popular Technical Reports.
© 2024 PatSnap. All rights reserved.Legal|Privacy policy|Modern Slavery Act Transparency Statement|Sitemap|About US| Contact US: help@patsnap.com