Layered integrated Gaussian process regression soft measurement modeling method
A Gaussian process regression and layered integration technology, applied in character and pattern recognition, complex mathematical operations, instruments, etc., can solve the problems of enhanced model diversity, underutilization, model structure uncertainty, etc., to reduce production costs , improve product quality, increase the effect of output
- Summary
- Abstract
- Description
- Claims
- Application Information
AI Technical Summary
Problems solved by technology
Method used
Image
Examples
Embodiment Construction
[0021] Combine below figure 1 Shown, the present invention is described in further detail:
[0022] Take the common chemical process—debutanizer process as an example. The experimental data comes from the debutanizer process to predict the concentration of butane in the bottom of the predicted product.
[0023] Step 1: Collect input and output data to form a historical training database.
[0024] Step 2: Estimate the parameters of the Gaussian mixture model (GMM) based on historical training data, and then divide the complete input and output training data into different operating stages. The GMM algorithm is:
[0025] GMM assumes that the data obey a mixture Gaussian distribution with unknown parameters. Given a training sample set X∈R n×m and y∈R n ×1 , where n is the number of sample points and m is the sample dimension. Assuming that the training sample X obeys the Gaussian mixture model of K components, the probability density can be expressed as:
[0026]
[0...
PUM
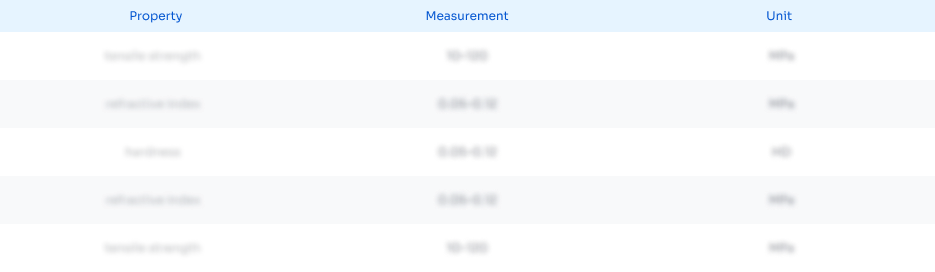
Abstract
Description
Claims
Application Information
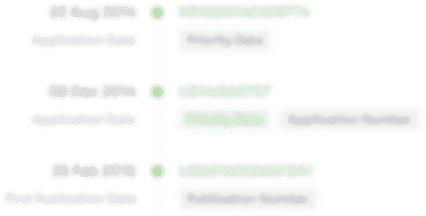
- Generate Ideas
- Intellectual Property
- Life Sciences
- Materials
- Tech Scout
- Unparalleled Data Quality
- Higher Quality Content
- 60% Fewer Hallucinations
Browse by: Latest US Patents, China's latest patents, Technical Efficacy Thesaurus, Application Domain, Technology Topic, Popular Technical Reports.
© 2025 PatSnap. All rights reserved.Legal|Privacy policy|Modern Slavery Act Transparency Statement|Sitemap|About US| Contact US: help@patsnap.com