Wind turbine generator bearing fault diagnosis method for multi-channel deep convolutional neural network
A deep convolution and neural network technology, applied in biological neural network models, mechanical bearing testing, neural architecture, etc., can solve problems such as low signal-to-noise ratio, poor diagnostic performance of fault samples, and difficulty in application, achieving good versatility and scalability, realize automatic feature learning, and avoid the effect of feature engineering
- Summary
- Abstract
- Description
- Claims
- Application Information
AI Technical Summary
Problems solved by technology
Method used
Image
Examples
Embodiment Construction
[0024] The specific implementation will be described in detail below in conjunction with the accompanying drawings.
[0025] A deep convolutional neural network-based fault diagnosis method for wind turbine bearings. The method includes diagnostic signal collection, diagnostic model establishment, model training and evaluation, and model application.
[0026] A wind turbine bearing fault diagnosis method based on deep convolutional neural network, such as figure 1 shown, including the following steps:
[0027] Step 1: Use the vibration acceleration sensor to simultaneously collect the high-frequency vibration acceleration signals of the driving end and the non-driving end of the test bearing in various states.
[0028] Step 2: Apply time-frequency analysis technology to the collected vibration signal to obtain the corresponding time-frequency spectrum.
[0029] Step 3: Establish a deep convolutional neural network diagnostic model, and use the time-frequency spectrum and bea...
PUM
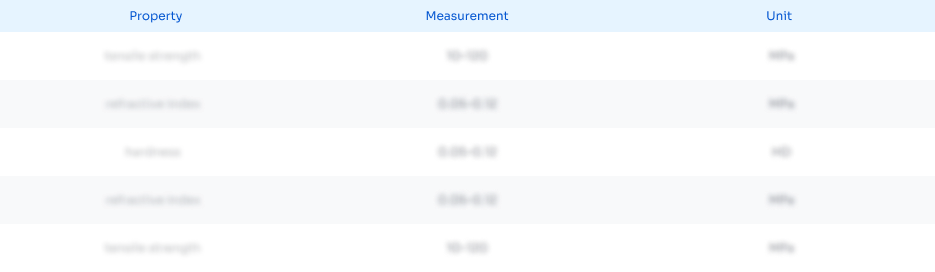
Abstract
Description
Claims
Application Information
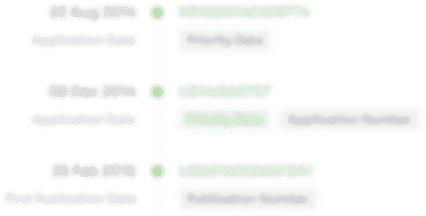
- R&D Engineer
- R&D Manager
- IP Professional
- Industry Leading Data Capabilities
- Powerful AI technology
- Patent DNA Extraction
Browse by: Latest US Patents, China's latest patents, Technical Efficacy Thesaurus, Application Domain, Technology Topic, Popular Technical Reports.
© 2024 PatSnap. All rights reserved.Legal|Privacy policy|Modern Slavery Act Transparency Statement|Sitemap|About US| Contact US: help@patsnap.com