An EEG feature extraction and classification method combining dae and cnn
An EEG signal and feature extraction technology, applied in neural learning methods, character and pattern recognition, instruments, etc., can solve the problems of high requirements on the number of EEG channels, complex parameter adjustment, and high time domain analysis overhead, and achieve simplification. The data collection process, the simplified network training process, and the effect of strong generalization ability
- Summary
- Abstract
- Description
- Claims
- Application Information
AI Technical Summary
Problems solved by technology
Method used
Image
Examples
Embodiment Construction
[0041] The technical solutions in the embodiments of the present invention will be described clearly and in detail below with reference to the drawings in the embodiments of the present invention. The described embodiments are only some of the embodiments of the invention.
[0042] The technical scheme that the present invention solves the problems of the technologies described above is:
[0043] (1) Select three healthy male subjects. There are 16 electrodes on the device, including reference electrodes CMS and DRL and 14 detachable electrodes, which are placed according to the international standard 10-20. The experimental environment is quiet and free from noise interference. The signal collection process is as follows: when t=0s, the experiment starts, and the subject keeps his mind awake and relaxed; when t=2s, a prompt sound appears, and the subject performs left hand or Right-hand imagination task; at t=4s, the subject ends the task according to the prompt tone, takes ...
PUM
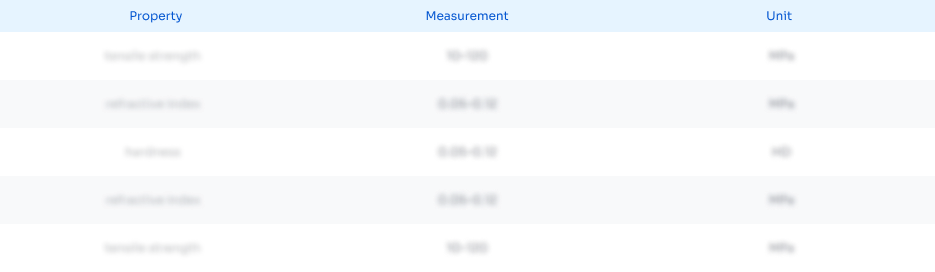
Abstract
Description
Claims
Application Information
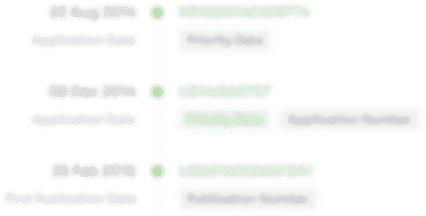
- R&D Engineer
- R&D Manager
- IP Professional
- Industry Leading Data Capabilities
- Powerful AI technology
- Patent DNA Extraction
Browse by: Latest US Patents, China's latest patents, Technical Efficacy Thesaurus, Application Domain, Technology Topic, Popular Technical Reports.
© 2024 PatSnap. All rights reserved.Legal|Privacy policy|Modern Slavery Act Transparency Statement|Sitemap|About US| Contact US: help@patsnap.com