Class mean kernel principal component fault diagnosis method based on combination kernel function
A combined kernel function and fault diagnosis technology, applied in the direction of instruments, electrical testing/monitoring, control/regulation systems, etc., can solve the problems of missed alarms, false alarms, complex model update process, etc., to achieve enhanced sensitivity and good tracking performance. Effect
- Summary
- Abstract
- Description
- Claims
- Application Information
AI Technical Summary
Problems solved by technology
Method used
Image
Examples
Embodiment 1
[0057] The TE process is a standard test (Benchmark) process proposed by Downs et al. The data used for the fault detection of the TE simulation system comes from http: / / brahms.scs.uiuc.edu. The TE process includes 41 measured variables and 12 controlled variables, and 21 typical faults are artificially set. The present invention takes the fault 13 in the TE process fault as an example, simulates and compares the influence of the traditional KPCA method and the class mean KPCA method of combined kernel functions on the fault detection rate. 480 sets of data are used as training data, and 960 sets of data are used as test data. Each set of observations contains 52 process variables. The training data is the data under normal working conditions, and the test data is the 161st sampling point under normal working conditions. 13 data. The simulation comparison results are as figure 1 , figure 2 with image 3 shown.
[0058] The fault detection rate corresponding to each fault...
Embodiment 2
[0064] Distillation tower is an indispensable and important device for chemical and oil refining enterprises. Once valve, concentration and other faults occur, it will bring great losses to the production of the enterprise. Therefore, the fault detection and diagnosis of distillation tower has become an important link in chemical production. Select 1000 data points from the solvent dehydration tower data, wherein the first 200 data points are used as modeling data, except that the simulated reflux flow drops sharply between the 400th and 500th data, all the other working conditions are running normally, verifying the present invention. The ability of the proposed method and the traditional class mean KPCA method to track the normal drift of the working conditions. Simulation results such as Figure 5 with Image 6 shown.
[0065] It can be seen that the mean-like kernel PCA method based on the combined kernel function has better performance in tracking the normal drift of wo...
PUM
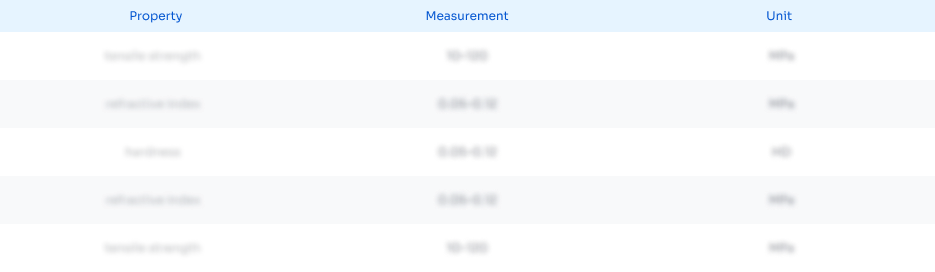
Abstract
Description
Claims
Application Information
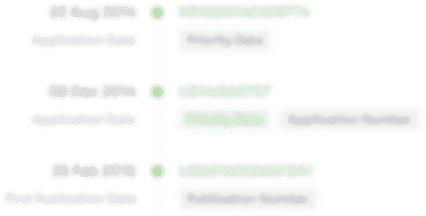
- R&D
- Intellectual Property
- Life Sciences
- Materials
- Tech Scout
- Unparalleled Data Quality
- Higher Quality Content
- 60% Fewer Hallucinations
Browse by: Latest US Patents, China's latest patents, Technical Efficacy Thesaurus, Application Domain, Technology Topic, Popular Technical Reports.
© 2025 PatSnap. All rights reserved.Legal|Privacy policy|Modern Slavery Act Transparency Statement|Sitemap|About US| Contact US: help@patsnap.com