A Method for Automatic Tracheal Tree Extraction from Chest CT Images
A CT image and tracheal tree technology, applied in the field of image processing based on medical images, can solve the problems of missing detailed information, restricting the accuracy of tracheal segmentation, and reducing the gray value
- Summary
- Abstract
- Description
- Claims
- Application Information
AI Technical Summary
Problems solved by technology
Method used
Image
Examples
Embodiment 1
[0121] The technical problem solved by this embodiment is to provide a method for automatically extracting the trachea tree from chest CT images, using an adaptive threshold to avoid manual interaction and parameter setting required by the prior art, so as to adapt to various imaging conditions and pathological conditions The tracheal tree segmentation task. Such as figure 1 As shown, the present invention provides a method for fully automatic extraction of trachea from chest CT images, and the technical scheme is as follows:
[0122] 101. Based on the chest CT image, the first type of tracheal branch of the tracheal tree, that is, the main trachea and the main bronchus, is obtained based on the 3D region growth segmentation method, wherein the main bronchus includes the left main bronchus and the right main bronchus;
[0123] 102. Based on the 3D region growth segmentation method, the obtained intermediate information of the main trachea, and the obtained intermediate inform...
Embodiment 2
[0129] The main trachea and main bronchus are surrounded by a relatively complete and bright tracheal wall and separated from the lung parenchyma. Extracting the main trachea and main bronchi from CT images does not need to consider leakage, and the difficulty is relatively low. Therefore, the general threshold 3D region growth segmentation method is used. Effective access to the main trachea and main bronchi.
[0130] In this embodiment, the first type of tracheal branch is obtained from chest CT images, including:
[0131] 1. Obtain the main trachea from chest CT images:
[0132]1011. Read in the chest CT image, in order to avoid the impact of CT image noise on the subsequent growth segmentation, perform Gaussian smoothing preprocessing on the chest CT image with a three-dimensional scale of σ=0.5mm;
[0133] 1012. Obtain a layer of CT images of the preprocessed chest CT image from the top of the chest to the bottom of the chest, and perform image binarization processing on...
Embodiment 3
[0151] In this embodiment, an adaptive threshold 3D region growth model and an adaptive threshold leakage model are established according to the acquired intermediate information such as the gray distribution of the main trachea and main bronchus, spatial scale, and segmentation process information.
[0152] The specific steps of establishing an adaptive threshold 3D region growing model are as follows:
[0153] 1021: Obtain an initial segmentation seed point set; the initial segmentation seed point set includes: all the seed points in the segmentation queue of the left main bronchus at the end of the iteration and all the seed points in the segmentation queue of the right main bronchus at the end of the iteration.
[0154] 1022: Obtain the grayscale threshold; in this embodiment, a certain grayscale threshold is set for the segmentation of the trachea tree to determine the upper limit of the trachea segmentation, and at the same time, pixels whose grayscale values exceed the...
PUM
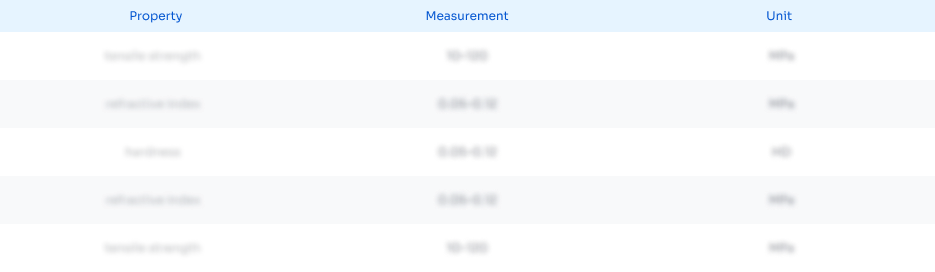
Abstract
Description
Claims
Application Information
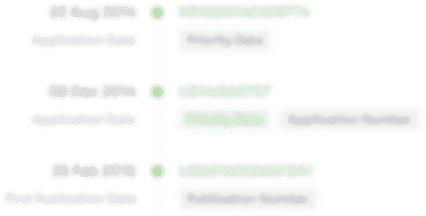
- R&D
- Intellectual Property
- Life Sciences
- Materials
- Tech Scout
- Unparalleled Data Quality
- Higher Quality Content
- 60% Fewer Hallucinations
Browse by: Latest US Patents, China's latest patents, Technical Efficacy Thesaurus, Application Domain, Technology Topic, Popular Technical Reports.
© 2025 PatSnap. All rights reserved.Legal|Privacy policy|Modern Slavery Act Transparency Statement|Sitemap|About US| Contact US: help@patsnap.com