LSTM deep learning model-based hydropower unit fault diagnosis method and system
A technology for hydroelectric generating units and fault diagnosis, applied in neural learning methods, motor generator testing, biological neural network models, etc. The effect of reduced computation, high efficiency and accuracy
- Summary
- Abstract
- Description
- Claims
- Application Information
AI Technical Summary
Problems solved by technology
Method used
Image
Examples
Embodiment 1
[0072] A method for diagnosing faults of hydropower units based on the LSTM deep learning model proposed in the embodiment of the present invention, refer to figure 1 The diagnostic model diagram shown, the specific implementation steps are as follows:
[0073] Step 1: Obtain the sampling values of the vibration signals of N different signal channels of the hydroelectric unit. The variational mode decomposition is performed on the vibration signal of each signal channel to obtain K decomposed IMF components.
[0074] Among them, the premise of VMD decomposition is to construct a variational problem. Assuming that each 'mode' has a finite bandwidth with a center frequency, the variational problem can be described as seeking K IMF components u k (t), the sum of the estimated bandwidths of each mode is minimized, and the constraint condition is that the sum of each mode is the original input signal. The construction process of the variational problem is as follows:
[0075] (...
Embodiment 2
[0134] The embodiment of the present invention also provides a hydroelectric unit fault diagnosis system based on the LSTM deep learning model, such as Figure 4 As shown, the system includes:
[0135] The IMF component acquisition unit is used to acquire the sampling sequences of N different signal channels of the hydroelectric unit, and perform variational mode decomposition on each time series to obtain K IMF components;
[0136] A training set and a set to be diagnosed acquisition unit, used for normalizing each IMF component, and constructing a corresponding training set and a set to be diagnosed;
[0137] A feature extraction unit is used to construct a long-short-term memory network model for the training set of each IMF component, and perform feature extraction for each intrinsic mode function through at least two layers of LSTM layers;
[0138] The training module is used to connect the outputs of K LSTM layers of the same signal channel to a Dense layer, and then conn...
PUM
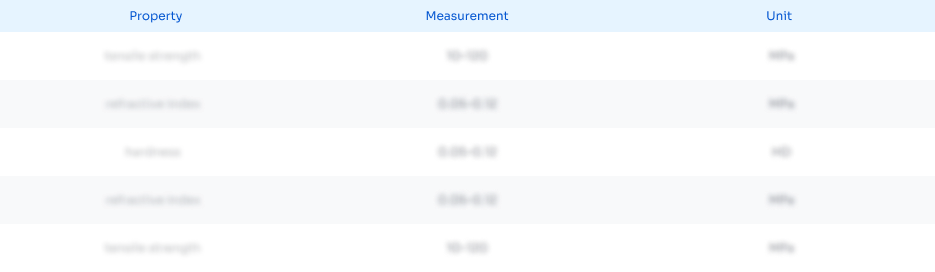
Abstract
Description
Claims
Application Information
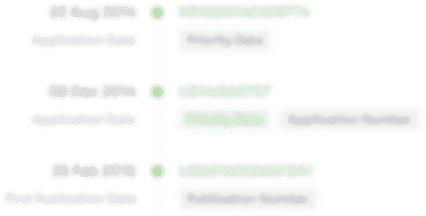
- R&D
- Intellectual Property
- Life Sciences
- Materials
- Tech Scout
- Unparalleled Data Quality
- Higher Quality Content
- 60% Fewer Hallucinations
Browse by: Latest US Patents, China's latest patents, Technical Efficacy Thesaurus, Application Domain, Technology Topic, Popular Technical Reports.
© 2025 PatSnap. All rights reserved.Legal|Privacy policy|Modern Slavery Act Transparency Statement|Sitemap|About US| Contact US: help@patsnap.com