Multistage target detection method and model based on CNN multistage feature fusion
A feature fusion and target detection technology, applied in the field of computational vision target detection, can solve the problems of increasing the target area of interest, increasing complexity, loss of feature information and position information, etc., to optimize the network structure, improve accuracy, and improve accuracy degree of effect
- Summary
- Abstract
- Description
- Claims
- Application Information
AI Technical Summary
Problems solved by technology
Method used
Image
Examples
Embodiment Construction
[0054] The main idea of the present invention is to fully consider the relationship between the scale size of the target in the image and the high-level and low-level feature maps, and further improve the detection of different-sized targets on the basis of balancing the speed and accuracy of target detection, so as to improve the detection of multiple types of targets. overall detection performance.
[0055] In order to make the technical solution of the present invention clearer and easier to understand, the present invention will be further described below in conjunction with the accompanying drawings and specific embodiments.
[0056] see figure 1 , the present invention provides the detection of objects of different sizes in the high-level and low-level feature maps in the image. In the existing general detection network, the target candidate frame is only extracted from the last layer of feature maps (high-level feature maps), such as figure 1 As shown in (a), when th...
PUM
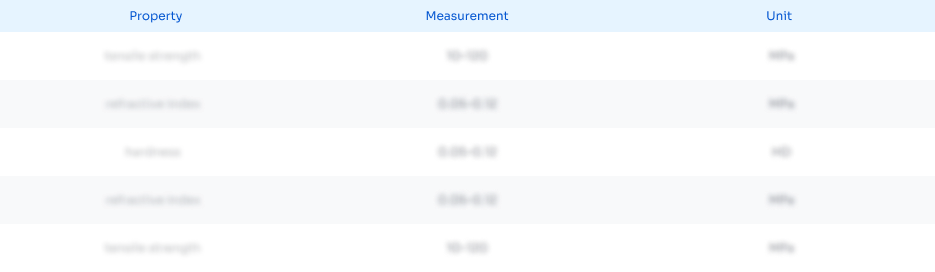
Abstract
Description
Claims
Application Information
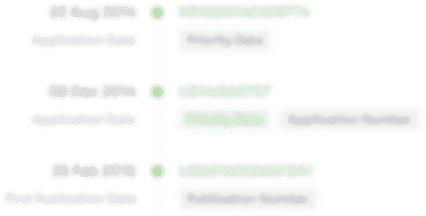
- R&D
- Intellectual Property
- Life Sciences
- Materials
- Tech Scout
- Unparalleled Data Quality
- Higher Quality Content
- 60% Fewer Hallucinations
Browse by: Latest US Patents, China's latest patents, Technical Efficacy Thesaurus, Application Domain, Technology Topic, Popular Technical Reports.
© 2025 PatSnap. All rights reserved.Legal|Privacy policy|Modern Slavery Act Transparency Statement|Sitemap|About US| Contact US: help@patsnap.com