Method for defense of attack of adversarial examples based on convolutional denoising auto-encoder
An adversarial sample and self-encoding technology, applied in the field of information security, can solve the problems of difficulty in fitting adversarial samples and clean samples at the same time, lack of interpretability, poor efficiency performance, etc., to reduce computational overhead, good interpretability, The effect of improving the classification accuracy
- Summary
- Abstract
- Description
- Claims
- Application Information
AI Technical Summary
Problems solved by technology
Method used
Image
Examples
Embodiment Construction
[0032] In order to illustrate the operation process of this method more vividly, we use the MNIST data set and the Cleverhans library to explain, but it is worth emphasizing that the present invention is not limited to the MNIST data set, but is generally applicable to any image data used for classification and recognition Set, and the implementation parameters need to be adjusted and modified according to the actual situation.
[0033] The MNIST data set is a handwritten digital data set constructed by Google Lab and the Courant Institute of New York University. The training set contains 60,000 digital images, and the test set contains 10,000 images. It is often used for prototype verification of image recognition algorithms; Cleverhans is An open source software library that provides a reference implementation of standard adversarial example construction, which can be used to develop more robust machine learning models. Cleverhans has a built-in FGSM (Fast Gradient Sign Meth...
PUM
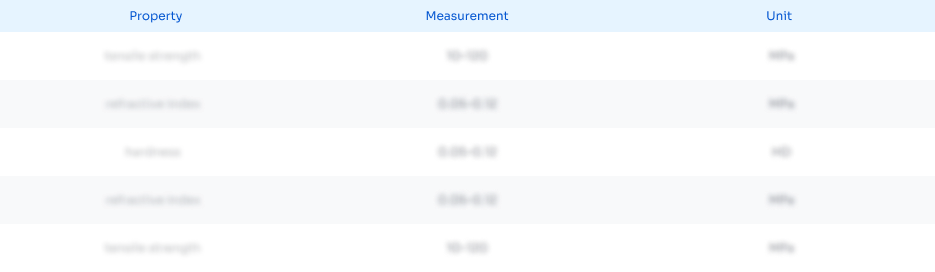
Abstract
Description
Claims
Application Information
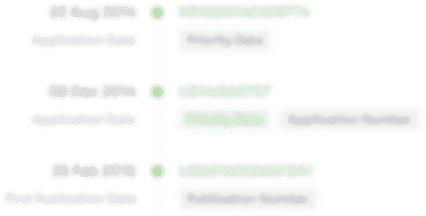
- R&D Engineer
- R&D Manager
- IP Professional
- Industry Leading Data Capabilities
- Powerful AI technology
- Patent DNA Extraction
Browse by: Latest US Patents, China's latest patents, Technical Efficacy Thesaurus, Application Domain, Technology Topic, Popular Technical Reports.
© 2024 PatSnap. All rights reserved.Legal|Privacy policy|Modern Slavery Act Transparency Statement|Sitemap|About US| Contact US: help@patsnap.com