Semi-supervised classification method capable of simultaneously learning affinity matrix and Laplacian regularized least square
A technique of least squares and correlation matrix, which is applied in character and pattern recognition, instruments, computer components, etc., can solve problems such as ineffective processing of foreign samples, label propagation methods not suitable for complex structured data sets, etc., to achieve improved performance effect
- Summary
- Abstract
- Description
- Claims
- Application Information
AI Technical Summary
Problems solved by technology
Method used
Image
Examples
Embodiment Construction
[0032] In order to make the object, technical solution and advantages of the present invention clearer, the present invention will be further described in detail below in conjunction with the accompanying drawings.
[0033] Such as Figure 1 to Figure 2 As shown, in the embodiment of the present invention, the present invention is a semi-supervised classification method that simultaneously learns the correlation matrix and the Laplacian regularized least squares, and the specific operating hardware and programming language of the method of the present invention are not limited. It can be written in any language, so other working modes will not be repeated here.
[0034] The embodiment of the present invention adopts a computer with Intel Xeon-E5 central processing unit and 16G byte internal memory, and has programmed the work program of the semi-supervised classification of simultaneously learning correlation matrix and Laplacian regularization least squares with Matlab langua...
PUM
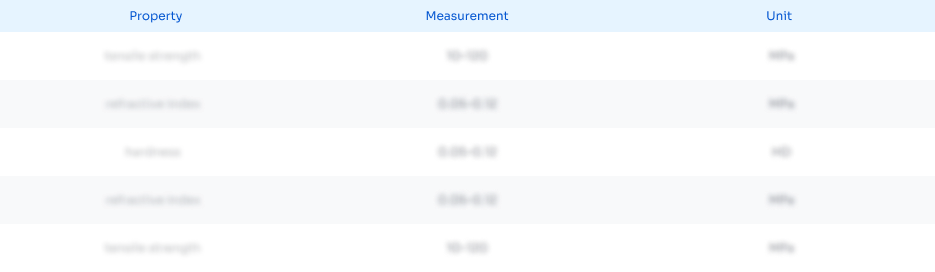
Abstract
Description
Claims
Application Information
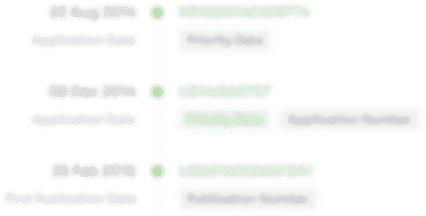
- Generate Ideas
- Intellectual Property
- Life Sciences
- Materials
- Tech Scout
- Unparalleled Data Quality
- Higher Quality Content
- 60% Fewer Hallucinations
Browse by: Latest US Patents, China's latest patents, Technical Efficacy Thesaurus, Application Domain, Technology Topic, Popular Technical Reports.
© 2025 PatSnap. All rights reserved.Legal|Privacy policy|Modern Slavery Act Transparency Statement|Sitemap|About US| Contact US: help@patsnap.com