Method for removing Poisson noise in mage based on non-local similarity low rank matrix
A non-local similarity and low-rank matrix technology, applied in the field of image denoising, can solve problems such as missing images, achieve good visual effects, and efficiently remove image noise
- Summary
- Abstract
- Description
- Claims
- Application Information
AI Technical Summary
Problems solved by technology
Method used
Image
Examples
Embodiment 1
[0046] Please refer to figure 1 , figure 1 The flow of the image denoising method is provided according to the implementation example of the present invention.
[0047] In this implementation example, the specific steps of the image removal Poisson noise method include the following steps:
[0048] 1) Analyzing noise: Assuming that the image noise obeys an independent Poisson distribution, according to the joint probability density function of Poisson distribution and the maximum likelihood principle, it is concluded that removing Poisson noise is equivalent to minimizing the KL-divergence function, because during the solution process, The instability of the value makes the effect of restoring the image unstable. Therefore, the prior knowledge of non-locally similar blocks in the image is used as a regularization term to stabilize the numerical solution.
[0049] 2) Modeling: First, assume that Poisson noise is independently distributed, so its joint probability density fun...
PUM
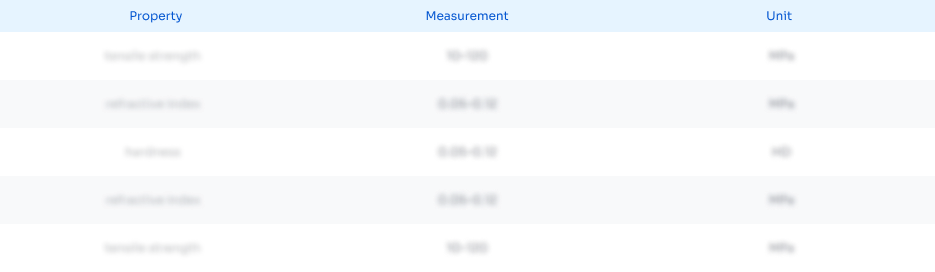
Abstract
Description
Claims
Application Information
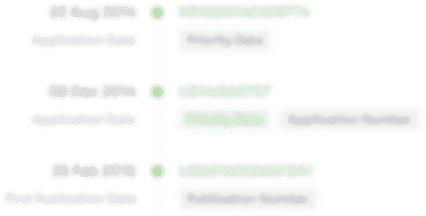
- R&D Engineer
- R&D Manager
- IP Professional
- Industry Leading Data Capabilities
- Powerful AI technology
- Patent DNA Extraction
Browse by: Latest US Patents, China's latest patents, Technical Efficacy Thesaurus, Application Domain, Technology Topic, Popular Technical Reports.
© 2024 PatSnap. All rights reserved.Legal|Privacy policy|Modern Slavery Act Transparency Statement|Sitemap|About US| Contact US: help@patsnap.com