Brain network oriented structured characteristic selection method
A feature selection method and brain network technology, applied in the field of structured feature selection for brain networks, can solve problems such as reducing classification performance, and achieve the effect of good performance
- Summary
- Abstract
- Description
- Claims
- Application Information
AI Technical Summary
Problems solved by technology
Method used
Image
Examples
Embodiment Construction
[0029] The present invention is described in further detail now in conjunction with accompanying drawing.
[0030] The present invention specifically adopts the following technical solutions:
[0031] Given a training sample set X=[x 1 , x 2 ..., x N ]∈R N*d , where x i Represents the feature vector of the i-th sample (such as: the feature vector composed of local measurements extracted from each network data), i=1,...,N, N represents the number of training samples, and d represents the feature dimension number. Let Y=[y 1 ,y 2 ...,y N ]∈R N represents a vector, where y i Represents the class label of the sample, for two-class classification problems, ie y i ∈ {+1, -1} (eg: +1 means patient, -1 means normal person).
[0032] In order to preserve the overall distribution information between samples, the following regularization term is introduced:
[0033]
[0034] Among them, g(x i )=w T x i is a linear mapping function, C=D-M is a Laplacian matrix, M=[M ij...
PUM
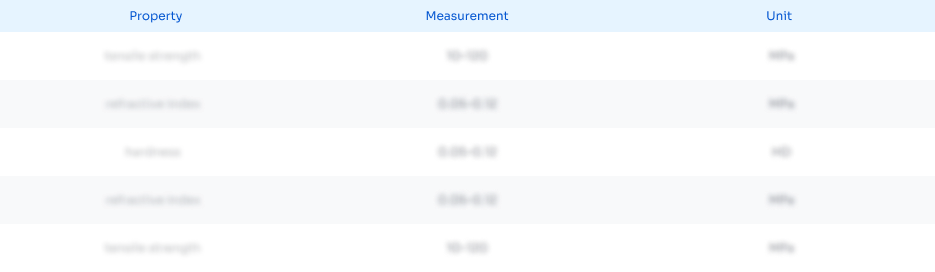
Abstract
Description
Claims
Application Information
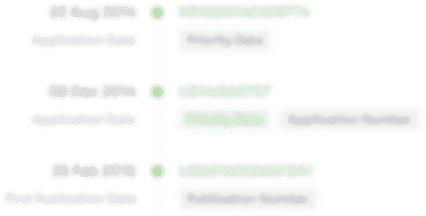
- R&D
- Intellectual Property
- Life Sciences
- Materials
- Tech Scout
- Unparalleled Data Quality
- Higher Quality Content
- 60% Fewer Hallucinations
Browse by: Latest US Patents, China's latest patents, Technical Efficacy Thesaurus, Application Domain, Technology Topic, Popular Technical Reports.
© 2025 PatSnap. All rights reserved.Legal|Privacy policy|Modern Slavery Act Transparency Statement|Sitemap|About US| Contact US: help@patsnap.com